It was a last-minute decision. The annual New York Film Festival was underway, and I had carefully studied its lineup. My list had four options: 1. Must see. 2. Should see. 3. See some time later on. And 4. Not really interested. The film playing that day was part of the second set. Sixty minutes before the film started, I decided to give it a shot. That meant driving for about 30 minutes, assuming no traffic hiccups, looking for a parking spot, walking-running to the theater, purchasing the ticket (not sold out yet, according to the website) and stepping into the large auditorium.
I was lucky traffic was not misbehaving that day. However, looking for a parking spot was a bit more complicated. I searched for a while, going around the same blocks several times. I was about to give up when a metered spot suddenly opened. I quickly parked and ran to the paying station to get a printed receipt I had to display on the car’s dashboard. I did that and ran two long blocks to the theater. The film was about to start when I finally sat down. Thanks to parking regulations, I only had two hours, so I had to forgo the planned after-film discussion and return to the car.
As I approached my vehicle, I saw a white piece of paper sticking out of the windshield, which meant I had been given a parking ticket. I was surprised as I had paid for the invaluable parking spot, and the clock indicated I still had two minutes to spare. But then I noticed I had placed the parking receipt upside down, so it was impossible to see the expiration time. And I was being asked to pay 60 dollars for my flagrant mistake. I began to ponder if I should dispute the alleged traffic violation. Back home, I decided to challenge it. In my mind, that meant I would have to go to a court and explain to a judge what had happened. I was certainly not looking forward to such an encounter, but on the other hand, I had not really done anything legally wrong.
In the back of the ticket, I saw a web address offered as one option for disputing the violation. I told my Open Source browser to drive me to that address. After entering the ticket number, I was asked to provide any evidence supporting my claim. I took a photo of my parking receipt and wrote a more extended version of the above events. I uploaded both to the site. I was also asked to send the original receipt and a printed version of my statement via snail mail. And the wait then started.
A few weeks later, I received an email indicating that a ruling on my case had been made. I was certainly not expecting that, as I assumed I would have to show my face in court at some point. Moreover, the message said the court had decided in my favor, thus voiding the ticket. “E-justice,” I loudly said to myself, happily sharing the news with my wife.
That took place almost 15 years ago and is an example of how public sector institutions have been deploying and using digital technologies since the advent of the private Internet. Initially branded as e-government , Digital Government (DG) has been around for over a quarter of a century. While its development is highly uneven across nations , most countries have DG policies, strategies, and/or initiatives.
One critical trait of the public sector’s embrace of new digital technologies is its relatively slow adoption pace. The sector is usually not a leader here. On the contrary, given its peculiar nature and structure, it always seems to be a late follower. After all, disbursing substantial public resources on programs and projects where unknowns prevail is not only risky but also irresponsible and unethical in the eyes of potential beneficiaries, stakeholders and citizens. Transparency and accountability should be the core drivers of such investments, digital or not .
Having done extensive work on DG in many countries, I have been able to identify three phases that characterize digital technologies’ deployment in the public sector: 1. Classic DG, 2. AI-driven deployments, And 3. GenAI-centered diffusion. I have already used plenty of digital real estate space to describe the classic phase, so there is no need to repeat myself here. The second phase started around 2015 after Deep Learning (DL) algorithms and models matured and conquered the application space. Classification, recommendation and predictive platforms were government favorites alongside chatbots that could directly interact with stakeholders desperately seeking government and public service information .
While the latter were indeed less than perfect, the harm created by the former clogged the headlines. Use and abuse in the criminal justice system and providing services such as health and insurance unveiled discriminatory practices that reflected bias within the DL algorithms and error rates unacceptable for public use. Part of the problem was the lack of human oversight and redressing mechanisms that those impacted by AI decisions could use to apply their cases . Unlike my e-justice example, there was no way to challenge a decision, correct or not. By the way, the same can be said about GenAI .
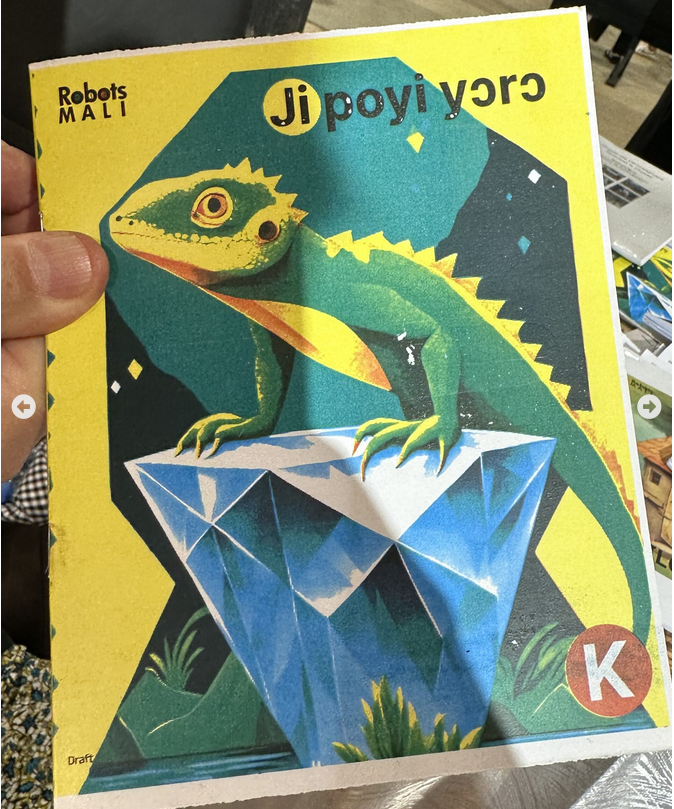
The final phase has just commenced. Successful GenAI models such as ChatGPT are still very young, albeit very loud and relatively successful. Governments are still pondering how to deploy them, if at all. That is certainly the case for those in the Global North, with a handful of Global South representatives doing the same. For example, countries such as Sierra Leone and Mali have already deployed GenAI to address educational gaps and challenges that may be very difficult to address otherwise .
Here, I can highlight three themes we should keep an eye on from here on in. First, AI’s shift from numerical to human-like text processing will definitely make a substantial difference within the public sector, too. That is undoubtedly the case so for all information-rich goods and services that cannot be easily mastered by “numeric” AI. Moreover, the fact that governments have already deployed chatbots based on older AI technology provides a clear entry point for replacing them with more sophisticated chatbots running on GenAI relatively quickly. The critical question here is not the technology per se but rather the institutional changes envisaged to ensure effective deployment and the new governance mechanisms to oversee implementation and address failures and glaring AI errors .
Second, we should not lose sight of the links between classic DG and AI developments. Most of the academic literature on AI in the public sector seems oblivious to this and seems to adopt a tabula rasa approach where AI is the only game in town . That is myopic, as AI’s resurgence results from the rapid evolution of Information and Communication Technologies (ICTs) that spearheaded big data, cloud and cluster computing, extraordinary computing capacity and low storage costs. AI depends on ICTs. However, AI can also change how the older ICTs operate. Indeed, it is a two-way street. As classic DG is based on ICTs, the question for policymakers is how AI, old and new, would impact existing digitalization initiatives and infostructures. GenAI has precisely the same effect. However, it can also affect how the public sector and its end users deploy and use older AI platforms. Complex dynamics across these three layers are surely to emerge. The public sector should thus be ready to tackle them.
The example of Sierra Leone and Mali pinpoint my third theme. Adopting state-of-the-art technologies is indeed possible in Global South countries where national income is exiguous, digital infrastructure is dispersed and expensive to access, and poverty is pervasive. And it is not limited to GenAI. I personally saw it in Dhaka, where a local ISP was deploying fiber optic cable for broadband connectivity in upper-class neighborhoods at a time (the mid-2010s) when I could not purchase such a connection in the US. Ten years later, something similar happened with Bitcoin and blockchain as many startups went to Global South countries (Honduras and Sierra Leone again!) to test their wares . The issue here is that such efforts, even if well-intentioned, have little short-term impact locally and are not sustainable in the long term. We should thus be mindful of neo-colonialism, intelligent or not .
Indeed, some things might change a lot. But quite a few others will not.
Raúl
References
{5575045:2Z46M5YY};{5575045:ASHBUC8U};{5575045:F94GQ7ZK},{5575045:RX76KXJA};{5575045:BDEV72R7},{5575045:292GSHQP},{5575045:I6HYF5TZ},{5575045:ZWNE2T2R},{5575045:B975SY7C},{5575045:SA7IH6PZ};{5575045:QLFYYDZK},{5575045:YQ5XQN74};{5575045:SAAA27EF},{5575045:GYEC9525};{5575045:423AELMK},{5575045:QVY6FGPR},{5575045:TS8JJASQ};{5575045:ZCQCDFM8},{5575045:VQ9CDYIF},{5575045:SUNPS9FG};{5575045:2DB233F6};{5575045:TFWCQ8PK};{5575045:TEI9JP8K}
apa
default
asc
no
1
12771
%7B%22status%22%3A%22success%22%2C%22updateneeded%22%3Afalse%2C%22instance%22%3A%22zotpress-a277ce6b1c3e20bf25a7021e9f47a0bb%22%2C%22meta%22%3A%7B%22request_last%22%3A0%2C%22request_next%22%3A0%2C%22used_cache%22%3Atrue%7D%2C%22data%22%3A%5B%7B%22key%22%3A%22BDEV72R7%22%2C%22library%22%3A%7B%22id%22%3A5575045%7D%2C%22meta%22%3A%7B%22creatorSummary%22%3A%22Cort%5Cu00e9s-Cediel%20et%20al.%22%2C%22parsedDate%22%3A%222023-10-01%22%2C%22numChildren%22%3A1%7D%2C%22bib%22%3A%22%3Cdiv%20class%3D%5C%22csl-bib-body%5C%22%20style%3D%5C%22line-height%3A%202%3B%20padding-left%3A%201em%3B%20text-indent%3A-1em%3B%5C%22%3E%5Cn%20%20%3Cdiv%20class%3D%5C%22csl-entry%5C%22%3ECort%26%23xE9%3Bs-Cediel%2C%20M.%20E.%2C%20Segura-Tinoco%2C%20A.%2C%20Cantador%2C%20I.%2C%20%26amp%3B%20Rodr%26%23xED%3Bguez%20Bol%26%23xED%3Bvar%2C%20M.%20P.%20%282023%29.%20Trends%20and%20challenges%20of%20e-government%20chatbots%3A%20Advances%20in%20exploring%20open%20government%20data%20and%20citizen%20participation%20content.%20%3Ci%3EGovernment%20Information%20Quarterly%3C%5C%2Fi%3E%2C%20%3Ci%3E40%3C%5C%2Fi%3E%284%29%2C%20101877.%20%3Ca%20href%3D%27https%3A%5C%2F%5C%2Fdoi.org%5C%2F10.1016%5C%2Fj.giq.2023.101877%27%3Ehttps%3A%5C%2F%5C%2Fdoi.org%5C%2F10.1016%5C%2Fj.giq.2023.101877%3C%5C%2Fa%3E%3C%5C%2Fdiv%3E%5Cn%3C%5C%2Fdiv%3E%22%2C%22data%22%3A%7B%22itemType%22%3A%22journalArticle%22%2C%22title%22%3A%22Trends%20and%20challenges%20of%20e-government%20chatbots%3A%20Advances%20in%20exploring%20open%20government%20data%20and%20citizen%20participation%20content%22%2C%22creators%22%3A%5B%7B%22creatorType%22%3A%22author%22%2C%22firstName%22%3A%22Mar%5Cu00eda%20E.%22%2C%22lastName%22%3A%22Cort%5Cu00e9s-Cediel%22%7D%2C%7B%22creatorType%22%3A%22author%22%2C%22firstName%22%3A%22Andr%5Cu00e9s%22%2C%22lastName%22%3A%22Segura-Tinoco%22%7D%2C%7B%22creatorType%22%3A%22author%22%2C%22firstName%22%3A%22Iv%5Cu00e1n%22%2C%22lastName%22%3A%22Cantador%22%7D%2C%7B%22creatorType%22%3A%22author%22%2C%22firstName%22%3A%22Manuel%20Pedro%22%2C%22lastName%22%3A%22Rodr%5Cu00edguez%20Bol%5Cu00edvar%22%7D%5D%2C%22abstractNote%22%3A%22In%20this%20paper%2C%20we%20propose%20a%20conceptual%20framework%20composed%20of%20a%20number%20of%20e-government%2C%20implementation%20and%20evaluation-oriented%20variables%2C%20with%20which%20we%20jointly%20analyze%20chatbots%20presented%20in%20the%20research%20literature%20and%20chatbots%20deployed%20as%20public%20services%20in%20Spain%20at%20national%2C%20regional%20and%20local%20levels.%20As%20a%20result%20of%20our%20holistic%20analysis%2C%20we%20identify%20and%20discuss%20current%20trends%20and%20challenges%20in%20the%20development%20and%20evaluation%20of%20chatbots%20in%20the%20public%20administration%20sector%2C%20such%20as%20focusing%20the%20use%20of%20the%20conversational%20agents%20on%20the%20search%20for%20government%20information%2C%20documents%20and%20services%20%5Cu2013leaving%20citizen%20consultation%20and%20collaboration%20aside%5Cu2013%2C%20and%20conducting%20preliminary%20evaluations%20of%20prototypes%20in%20limited%20studies%2C%20lacking%20experiments%20on%20deployed%20systems%2C%20with%20metrics%20beyond%20effectiveness%20and%20usability%20%5Cu2013e.g.%2C%20metrics%20related%20to%20the%20generation%20of%20public%20values.%20Addressing%20some%20of%20the%20identified%20challenges%2C%20we%20build%20and%20evaluate%20two%20novel%20chatbots%20that%20present%20advances%20in%20the%20access%20to%20open%20government%20data%20and%20citizen%20participation%20content.%20Moreover%2C%20we%20come%20up%20with%20additional%2C%20potential%20research%20lines%20that%20may%20be%20considered%20in%20the%20future%20for%20a%20new%20generation%20of%20e-government%20chatbots.%22%2C%22date%22%3A%222023-10-01%22%2C%22language%22%3A%22%22%2C%22DOI%22%3A%2210.1016%5C%2Fj.giq.2023.101877%22%2C%22ISSN%22%3A%220740-624X%22%2C%22url%22%3A%22https%3A%5C%2F%5C%2Fwww.sciencedirect.com%5C%2Fscience%5C%2Farticle%5C%2Fpii%5C%2FS0740624X23000771%22%2C%22collections%22%3A%5B%22ILXVZG9Z%22%5D%2C%22dateModified%22%3A%222024-03-08T23%3A04%3A18Z%22%7D%7D%2C%7B%22key%22%3A%22QLFYYDZK%22%2C%22library%22%3A%7B%22id%22%3A5575045%7D%2C%22meta%22%3A%7B%22creatorSummary%22%3A%22Alon-Barkat%20and%20Busuioc%22%2C%22parsedDate%22%3A%222023-01-01%22%2C%22numChildren%22%3A1%7D%2C%22bib%22%3A%22%3Cdiv%20class%3D%5C%22csl-bib-body%5C%22%20style%3D%5C%22line-height%3A%202%3B%20padding-left%3A%201em%3B%20text-indent%3A-1em%3B%5C%22%3E%5Cn%20%20%3Cdiv%20class%3D%5C%22csl-entry%5C%22%3EAlon-Barkat%2C%20S.%2C%20%26amp%3B%20Busuioc%2C%20M.%20%282023%29.%20Human%26%23×2013%3BAI%20Interactions%20in%20Public%20Sector%20Decision%20Making%3A%20%26%23x201C%3BAutomation%20Bias%26%23x201D%3B%20and%20%26%23x201C%3BSelective%20Adherence%26%23x201D%3B%20to%20Algorithmic%20Advice.%20%3Ci%3EJournal%20of%20Public%20Administration%20Research%20and%20Theory%3C%5C%2Fi%3E%2C%20%3Ci%3E33%3C%5C%2Fi%3E%281%29%2C%20153%26%23×2013%3B169.%20%3Ca%20href%3D%27https%3A%5C%2F%5C%2Fdoi.org%5C%2F10.1093%5C%2Fjopart%5C%2Fmuac007%27%3Ehttps%3A%5C%2F%5C%2Fdoi.org%5C%2F10.1093%5C%2Fjopart%5C%2Fmuac007%3C%5C%2Fa%3E%3C%5C%2Fdiv%3E%5Cn%3C%5C%2Fdiv%3E%22%2C%22data%22%3A%7B%22itemType%22%3A%22journalArticle%22%2C%22title%22%3A%22Human%5Cu2013AI%20Interactions%20in%20Public%20Sector%20Decision%20Making%3A%20%5Cu201cAutomation%20Bias%5Cu201d%20and%20%5Cu201cSelective%20Adherence%5Cu201d%20to%20Algorithmic%20Advice%22%2C%22creators%22%3A%5B%7B%22creatorType%22%3A%22author%22%2C%22firstName%22%3A%22Saar%22%2C%22lastName%22%3A%22Alon-Barkat%22%7D%2C%7B%22creatorType%22%3A%22author%22%2C%22firstName%22%3A%22Madalina%22%2C%22lastName%22%3A%22Busuioc%22%7D%5D%2C%22abstractNote%22%3A%22Artificial%20intelligence%20algorithms%20are%20increasingly%20adopted%20as%20decisional%20aides%20by%20public%20bodies%2C%20with%20the%20promise%20of%20overcoming%20biases%20of%20human%20decision-makers.%20At%20the%20same%20time%2C%20they%20may%20introduce%20new%20biases%20in%20the%20human%5Cu2013algorithm%20interaction.%20Drawing%20on%20psychology%20and%20public%20administration%20literatures%2C%20we%20investigate%20two%20key%20biases%3A%20overreliance%20on%20algorithmic%20advice%20even%20in%20the%20face%20of%20%5Cu201cwarning%20signals%5Cu201d%20from%20other%20sources%20%28automation%20bias%29%2C%20and%20selective%20adoption%20of%20algorithmic%20advice%20when%20this%20corresponds%20to%20stereotypes%20%28selective%20adherence%29.%20We%20assess%20these%20via%20three%20experimental%20studies%20conducted%20in%20the%20Netherlands%3A%20In%20study%201%20%28N%20%3D%20605%29%2C%20we%20test%20automation%20bias%20by%20exploring%20participants%5Cu2019%20adherence%20to%20an%20algorithmic%20prediction%20compared%20to%20an%20equivalent%20human-expert%20prediction.%20We%20do%20not%20find%20evidence%20for%20automation%20bias.%20In%20study%202%20%28N%20%3D%20904%29%2C%20we%20replicate%20these%20findings%2C%20and%20also%20test%20selective%20adherence.%20We%20find%20a%20stronger%20propensity%20for%20adherence%20when%20the%20advice%20is%20aligned%20with%20group%20stereotypes%2C%20with%20no%20significant%20differences%20between%20algorithmic%20and%20human-expert%20advice.%20In%20study%203%20%28N%20%3D%201%2C345%29%2C%20we%20replicate%20our%20design%20with%20a%20sample%20of%20civil%20servants.%20This%20study%20was%20conducted%20shortly%20after%20a%20major%20scandal%20involving%20public%20authorities%5Cu2019%20reliance%20on%20an%20algorithm%20with%20discriminatory%20outcomes%20%28the%20%5Cu201cchildcare%20benefits%20scandal%5Cu201d%29.%20The%20scandal%20is%20itself%20illustrative%20of%20our%20theory%20and%20patterns%20diagnosed%20empirically%20in%20our%20experiment%2C%20yet%20in%20our%20study%203%2C%20while%20supporting%20our%20prior%20findings%20as%20to%20automation%20bias%2C%20we%20do%20not%20find%20patterns%20of%20selective%20adherence.%20We%20suggest%20this%20is%20driven%20by%20bureaucrats%5Cu2019%20enhanced%20awareness%20of%20discrimination%20and%20algorithmic%20biases%20in%20the%20aftermath%20of%20the%20scandal.%20We%20discuss%20the%20implications%20of%20our%20findings%20for%20public%20sector%20decision%20making%20in%20the%20age%20of%20automation.%20Overall%2C%20our%20study%20speaks%20to%20potential%20negative%20effects%20of%20automation%20of%20the%20administrative%20state%20for%20already%20vulnerable%20and%20disadvantaged%20citizens.%22%2C%22date%22%3A%222023-01-01%22%2C%22language%22%3A%22%22%2C%22DOI%22%3A%2210.1093%5C%2Fjopart%5C%2Fmuac007%22%2C%22ISSN%22%3A%221053-1858%22%2C%22url%22%3A%22https%3A%5C%2F%5C%2Fdoi.org%5C%2F10.1093%5C%2Fjopart%5C%2Fmuac007%22%2C%22collections%22%3A%5B%22ILXVZG9Z%22%5D%2C%22dateModified%22%3A%222024-03-08T23%3A04%3A16Z%22%7D%7D%2C%7B%22key%22%3A%22292GSHQP%22%2C%22library%22%3A%7B%22id%22%3A5575045%7D%2C%22meta%22%3A%7B%22creatorSummary%22%3A%22Maragno%20et%20al.%22%2C%22parsedDate%22%3A%222023-11-02%22%2C%22numChildren%22%3A1%7D%2C%22bib%22%3A%22%3Cdiv%20class%3D%5C%22csl-bib-body%5C%22%20style%3D%5C%22line-height%3A%202%3B%20padding-left%3A%201em%3B%20text-indent%3A-1em%3B%5C%22%3E%5Cn%20%20%3Cdiv%20class%3D%5C%22csl-entry%5C%22%3EMaragno%2C%20G.%2C%20Tangi%2C%20L.%2C%20Gastaldi%2C%20L.%2C%20%26amp%3B%20Benedetti%2C%20M.%20%282023%29.%20AI%20as%20an%20organizational%20agent%20to%20nurture%3A%20effectively%20introducing%20chatbots%20in%20public%20entities.%20%3Ci%3EPublic%20Management%20Review%3C%5C%2Fi%3E%2C%20%3Ci%3E25%3C%5C%2Fi%3E%2811%29%2C%202135%26%23×2013%3B2165.%20%3Ca%20href%3D%27https%3A%5C%2F%5C%2Fdoi.org%5C%2F10.1080%5C%2F14719037.2022.2063935%27%3Ehttps%3A%5C%2F%5C%2Fdoi.org%5C%2F10.1080%5C%2F14719037.2022.2063935%3C%5C%2Fa%3E%3C%5C%2Fdiv%3E%5Cn%3C%5C%2Fdiv%3E%22%2C%22data%22%3A%7B%22itemType%22%3A%22journalArticle%22%2C%22title%22%3A%22AI%20as%20an%20organizational%20agent%20to%20nurture%3A%20effectively%20introducing%20chatbots%20in%20public%20entities%22%2C%22creators%22%3A%5B%7B%22creatorType%22%3A%22author%22%2C%22firstName%22%3A%22Giulia%22%2C%22lastName%22%3A%22Maragno%22%7D%2C%7B%22creatorType%22%3A%22author%22%2C%22firstName%22%3A%22Luca%22%2C%22lastName%22%3A%22Tangi%22%7D%2C%7B%22creatorType%22%3A%22author%22%2C%22firstName%22%3A%22Luca%22%2C%22lastName%22%3A%22Gastaldi%22%7D%2C%7B%22creatorType%22%3A%22author%22%2C%22firstName%22%3A%22Michele%22%2C%22lastName%22%3A%22Benedetti%22%7D%5D%2C%22abstractNote%22%3A%22We%20investigate%20how%20AI%20introduction%20affects%20public%20entities%20at%20the%20micro-level%2C%20hence%20the%20roles%2C%20competences%20and%20tasks%20of%20the%20agents%20involved.%20In%20doing%20so%2C%20we%20rely%20on%20the%20organizational%20design%20theory%20and%20we%20focus%20on%20a%20specific%20AI%20solution%20%28chatbot%29%20implemented%20within%20a%20defined%20microstructure%2C%20the%20customer%20service%20department.%20Using%20data%20collected%20through%20six%20exploratory%20case%20studies%2C%20we%20show%20how%20the%20creation%20of%20an%20AI%20team%20becomes%20a%20novel%20form%20of%20organizing%20that%20solves%20the%20universal%20problems%20of%20organizing.%20Results%20confirm%20that%20AI%20implementation%20is%20a%20complex%20organizational%20challenge%20and%20suggest%20that%20artificial%20agents%20act%20similarly%20to%20human%20ones.%22%2C%22date%22%3A%222023-11-02%22%2C%22language%22%3A%22%22%2C%22DOI%22%3A%2210.1080%5C%2F14719037.2022.2063935%22%2C%22ISSN%22%3A%221471-9037%22%2C%22url%22%3A%22https%3A%5C%2F%5C%2Fdoi.org%5C%2F10.1080%5C%2F14719037.2022.2063935%22%2C%22collections%22%3A%5B%22ILXVZG9Z%22%5D%2C%22dateModified%22%3A%222024-03-08T23%3A04%3A15Z%22%7D%7D%2C%7B%22key%22%3A%22SUNPS9FG%22%2C%22library%22%3A%7B%22id%22%3A5575045%7D%2C%22meta%22%3A%7B%22creatorSummary%22%3A%22Medaglia%20and%20Tangi%22%2C%22parsedDate%22%3A%222022-11-18%22%2C%22numChildren%22%3A0%7D%2C%22bib%22%3A%22%3Cdiv%20class%3D%5C%22csl-bib-body%5C%22%20style%3D%5C%22line-height%3A%202%3B%20padding-left%3A%201em%3B%20text-indent%3A-1em%3B%5C%22%3E%5Cn%20%20%3Cdiv%20class%3D%5C%22csl-entry%5C%22%3EMedaglia%2C%20R.%2C%20%26amp%3B%20Tangi%2C%20L.%20%282022%29.%20The%20adoption%20of%20Artificial%20Intelligence%20in%20the%20public%20sector%20in%20Europe%3A%20drivers%2C%20features%2C%20and%20impacts.%20%3Ci%3EProceedings%20of%20the%2015th%20International%20Conference%20on%20Theory%20and%20Practice%20of%20Electronic%20Governance%3C%5C%2Fi%3E%2C%2010%26%23×2013%3B18.%20%3Ca%20href%3D%27https%3A%5C%2F%5C%2Fdoi.org%5C%2F10.1145%5C%2F3560107.3560110%27%3Ehttps%3A%5C%2F%5C%2Fdoi.org%5C%2F10.1145%5C%2F3560107.3560110%3C%5C%2Fa%3E%3C%5C%2Fdiv%3E%5Cn%3C%5C%2Fdiv%3E%22%2C%22data%22%3A%7B%22itemType%22%3A%22conferencePaper%22%2C%22title%22%3A%22The%20adoption%20of%20Artificial%20Intelligence%20in%20the%20public%20sector%20in%20Europe%3A%20drivers%2C%20features%2C%20and%20impacts%22%2C%22creators%22%3A%5B%7B%22creatorType%22%3A%22author%22%2C%22firstName%22%3A%22Rony%22%2C%22lastName%22%3A%22Medaglia%22%7D%2C%7B%22creatorType%22%3A%22author%22%2C%22firstName%22%3A%22Luca%22%2C%22lastName%22%3A%22Tangi%22%7D%5D%2C%22abstractNote%22%3A%22This%20paper%20presents%20the%20findings%20of%20an%20online%20survey%20carried%20out%20as%20part%20of%20AI%20Watch%2C%20the%20European%20Commission%20knowledge%20service%20to%20monitor%20the%20development%2C%20uptake%20and%20impact%20of%20Artificial%20Intelligence%20%28AI%29%20for%20Europe.%20The%20survey%20was%20addressed%20at%20practitioners%20of%20public%20administrations%20at%20central%2C%20regional%2C%20and%20local%20level%20and%20aimed%20to%20compile%20a%20collection%20of%20cases%20of%20AI-enabled%20solutions%20used%20by%20public%20sector%20administrations.%20It%20analyses%20the%20drivers%2C%20obstacles%2C%20opportunities%2C%20and%20influencing%20factors%20of%20AI%20adoption%20and%20use%20by%20European%20public%20sector%20administrations%2C%20and%20identifies%20the%20perceived%20impacts%20of%20AI-enabled%20solutions%20on%20the%20different%20beneficiaries%5C%2Fusers%20of%20services%20provided%20by%20public%20sector%20administrations.%20Findings%20from%2062%20respondents%20show%20that%20there%20is%20a%20wide%20array%20of%20AI%20initiatives%20in%20the%20public%20sector%20in%20European%20Member%20States%20moving%20beyond%20the%20pilot%20stage%2C%20that%20there%20is%20lack%20of%20citizen%20involvement%20in%20the%20design%20of%20AI%20services%2C%20low%20digital%20literacy%20of%20employees%20using%20AI%20systems%2C%20and%20that%20the%20disrupting%20effect%20that%20AI%20is%20expected%20to%20have%20in%20the%20public%20sector%20is%20still%20not%20mirrored%20in%20concrete%20large-scale%20AI%20projects%20with%20wide%20impact%20on%20public%20affairs.%22%2C%22date%22%3A%22November%2018%2C%202022%22%2C%22proceedingsTitle%22%3A%22Proceedings%20of%20the%2015th%20International%20Conference%20on%20Theory%20and%20Practice%20of%20Electronic%20Governance%22%2C%22conferenceName%22%3A%22%22%2C%22language%22%3A%22%22%2C%22DOI%22%3A%2210.1145%5C%2F3560107.3560110%22%2C%22ISBN%22%3A%22978-1-4503-9635-6%22%2C%22url%22%3A%22https%3A%5C%2F%5C%2Fdoi.org%5C%2F10.1145%5C%2F3560107.3560110%22%2C%22collections%22%3A%5B%22ILXVZG9Z%22%5D%2C%22dateModified%22%3A%222024-03-08T23%3A04%3A15Z%22%7D%7D%2C%7B%22key%22%3A%22ZCQCDFM8%22%2C%22library%22%3A%7B%22id%22%3A5575045%7D%2C%22meta%22%3A%7B%22creatorSummary%22%3A%22Valle-Cruz%20et%20al.%22%2C%22parsedDate%22%3A%222023-08-08%22%2C%22numChildren%22%3A2%7D%2C%22bib%22%3A%22%3Cdiv%20class%3D%5C%22csl-bib-body%5C%22%20style%3D%5C%22line-height%3A%202%3B%20padding-left%3A%201em%3B%20text-indent%3A-1em%3B%5C%22%3E%5Cn%20%20%3Cdiv%20class%3D%5C%22csl-entry%5C%22%3EValle-Cruz%2C%20D.%2C%20Garc%26%23xED%3Ba-Contreras%2C%20R.%2C%20%26amp%3B%20Gil-Garcia%2C%20J.%20R.%20%282023%29.%20Exploring%20the%20negative%20impacts%20of%20artificial%20intelligence%20in%20government%3A%20the%20dark%20side%20of%20intelligent%20algorithms%20and%20cognitive%20machines.%20%3Ci%3EInternational%20Review%20of%20Administrative%20Sciences%3C%5C%2Fi%3E%2C%2000208523231187051.%20%3Ca%20href%3D%27https%3A%5C%2F%5C%2Fdoi.org%5C%2F10.1177%5C%2F00208523231187051%27%3Ehttps%3A%5C%2F%5C%2Fdoi.org%5C%2F10.1177%5C%2F00208523231187051%3C%5C%2Fa%3E%3C%5C%2Fdiv%3E%5Cn%3C%5C%2Fdiv%3E%22%2C%22data%22%3A%7B%22itemType%22%3A%22journalArticle%22%2C%22title%22%3A%22Exploring%20the%20negative%20impacts%20of%20artificial%20intelligence%20in%20government%3A%20the%20dark%20side%20of%20intelligent%20algorithms%20and%20cognitive%20machines%22%2C%22creators%22%3A%5B%7B%22creatorType%22%3A%22author%22%2C%22firstName%22%3A%22David%22%2C%22lastName%22%3A%22Valle-Cruz%22%7D%2C%7B%22creatorType%22%3A%22author%22%2C%22firstName%22%3A%22Rigoberto%22%2C%22lastName%22%3A%22Garc%5Cu00eda-Contreras%22%7D%2C%7B%22creatorType%22%3A%22author%22%2C%22firstName%22%3A%22J.%20Ramon%22%2C%22lastName%22%3A%22Gil-Garcia%22%7D%5D%2C%22abstractNote%22%3A%22This%20research%20proposes%20a%20framework%20for%20the%20negative%20impacts%20of%20artificial%20intelligence%20%28AI%29%20in%20government%20by%20classifying%2014%20topics%20of%20its%20dark%20side%20into%20five%20socio%20technical%20categories.%20The%20framework%20is%20based%20on%20a%20systematic%20literature%20review%20and%20highlights%20that%20the%20dark%20side%20is%20predominantly%20driven%20by%20political%2C%20legal%2C%20and%20institutional%20aspects%2C%20but%20it%20is%20also%20influenced%20by%20data%20and%20technology.%20Lack%20of%20understanding%20of%20AI%20outcomes%2C%20biases%2C%20and%20errors%2C%20as%20well%20as%20manipulation%20of%20intelligent%20algorithms%20and%20cognitive%20machines%20are%20contributing%20factors.%20The%20public%20sector%20should%20create%20knowledge%20about%20AI%20from%20an%20ethical%2C%20inclusive%2C%20and%20strategic%20perspective%2C%20involving%20experts%20from%20different%20areas.%5CnPoints%20for%20practitioners%5CnGovernment%20officials%20and%20other%20decision-makers%20should%20be%20aware%20of%20the%20potential%20benefits%20of%20artificial%20intelligence%2C%20but%20also%20of%20the%20dark%20side%2C%20and%20try%20to%20avoid%20those%20potential%20negative%20consequences.%22%2C%22date%22%3A%222023-08-08%22%2C%22language%22%3A%22en%22%2C%22DOI%22%3A%2210.1177%5C%2F00208523231187051%22%2C%22ISSN%22%3A%220020-8523%22%2C%22url%22%3A%22https%3A%5C%2F%5C%2Fdoi.org%5C%2F10.1177%5C%2F00208523231187051%22%2C%22collections%22%3A%5B%22ILXVZG9Z%22%5D%2C%22dateModified%22%3A%222024-03-08T23%3A04%3A12Z%22%7D%7D%2C%7B%22key%22%3A%22VQ9CDYIF%22%2C%22library%22%3A%7B%22id%22%3A5575045%7D%2C%22meta%22%3A%7B%22creatorSummary%22%3A%22Medaglia%20et%20al.%22%2C%22parsedDate%22%3A%222023-02-01%22%2C%22numChildren%22%3A1%7D%2C%22bib%22%3A%22%3Cdiv%20class%3D%5C%22csl-bib-body%5C%22%20style%3D%5C%22line-height%3A%202%3B%20padding-left%3A%201em%3B%20text-indent%3A-1em%3B%5C%22%3E%5Cn%20%20%3Cdiv%20class%3D%5C%22csl-entry%5C%22%3EMedaglia%2C%20R.%2C%20Gil-Garcia%2C%20J.%20R.%2C%20%26amp%3B%20Pardo%2C%20T.%20A.%20%282023%29.%20Artificial%20Intelligence%20in%20Government%3A%20Taking%20Stock%20and%20Moving%20Forward.%20%3Ci%3ESocial%20Science%20Computer%20Review%3C%5C%2Fi%3E%2C%20%3Ci%3E41%3C%5C%2Fi%3E%281%29%2C%20123%26%23×2013%3B140.%20%3Ca%20href%3D%27https%3A%5C%2F%5C%2Fdoi.org%5C%2F10.1177%5C%2F08944393211034087%27%3Ehttps%3A%5C%2F%5C%2Fdoi.org%5C%2F10.1177%5C%2F08944393211034087%3C%5C%2Fa%3E%3C%5C%2Fdiv%3E%5Cn%3C%5C%2Fdiv%3E%22%2C%22data%22%3A%7B%22itemType%22%3A%22journalArticle%22%2C%22title%22%3A%22Artificial%20Intelligence%20in%20Government%3A%20Taking%20Stock%20and%20Moving%20Forward%22%2C%22creators%22%3A%5B%7B%22creatorType%22%3A%22author%22%2C%22firstName%22%3A%22Rony%22%2C%22lastName%22%3A%22Medaglia%22%7D%2C%7B%22creatorType%22%3A%22author%22%2C%22firstName%22%3A%22J.%20Ramon%22%2C%22lastName%22%3A%22Gil-Garcia%22%7D%2C%7B%22creatorType%22%3A%22author%22%2C%22firstName%22%3A%22Theresa%20A.%22%2C%22lastName%22%3A%22Pardo%22%7D%5D%2C%22abstractNote%22%3A%22The%20use%20of%20artificial%20intelligence%20%28AI%29%20applications%20in%20government%20is%20receiving%20increasing%20attention%20from%20global%20research%20and%20practice%20communities.%20This%20article%2C%20introducing%20a%20Special%20Issue%20on%20Artificial%20Intelligence%20in%20Government%20published%20in%20the%20Social%20Science%20Computer%20Review%2C%20presents%20an%20overview%20of%20some%20of%20the%20main%20policy%20initiatives%20across%20the%20world%20in%20relation%20to%20AI%20in%20government%20and%20discusses%20the%20state%20of%20the%20art%20of%20existing%20research.%20Based%20on%20an%20analysis%20of%20current%20trends%20in%20research%20and%20practice%2C%20we%20highlight%20four%20areas%20to%20be%20the%20focus%20of%20future%20research%20on%20AI%20in%20government%3A%20governance%20of%20AI%2C%20trustworthy%20AI%2C%20impact%20assessment%20methodologies%2C%20and%20data%20governance.%22%2C%22date%22%3A%222023-02-01%22%2C%22language%22%3A%22en%22%2C%22DOI%22%3A%2210.1177%5C%2F08944393211034087%22%2C%22ISSN%22%3A%220894-4393%22%2C%22url%22%3A%22https%3A%5C%2F%5C%2Fdoi.org%5C%2F10.1177%5C%2F08944393211034087%22%2C%22collections%22%3A%5B%22ILXVZG9Z%22%5D%2C%22dateModified%22%3A%222024-03-08T23%3A04%3A11Z%22%7D%7D%2C%7B%22key%22%3A%22I6HYF5TZ%22%2C%22library%22%3A%7B%22id%22%3A5575045%7D%2C%22meta%22%3A%7B%22creatorSummary%22%3A%22van%20Noordt%20and%20Misuraca%22%2C%22parsedDate%22%3A%222019%22%2C%22numChildren%22%3A1%7D%2C%22bib%22%3A%22%3Cdiv%20class%3D%5C%22csl-bib-body%5C%22%20style%3D%5C%22line-height%3A%202%3B%20padding-left%3A%201em%3B%20text-indent%3A-1em%3B%5C%22%3E%5Cn%20%20%3Cdiv%20class%3D%5C%22csl-entry%5C%22%3Evan%20Noordt%2C%20C.%2C%20%26amp%3B%20Misuraca%2C%20G.%20%282019%29.%20New%20Wine%20in%20Old%20Bottles%3A%20Chatbots%20in%20Government.%20In%20P.%20Panagiotopoulos%2C%20N.%20Edelmann%2C%20O.%20Glassey%2C%20G.%20Misuraca%2C%20P.%20Parycek%2C%20T.%20Lampoltshammer%2C%20%26amp%3B%20B.%20Re%20%28Eds.%29%2C%20%3Ci%3EElectronic%20Participation%3C%5C%2Fi%3E%20%28pp.%2049%26%23×2013%3B59%29.%20Springer%20International%20Publishing.%20%3Ca%20href%3D%27https%3A%5C%2F%5C%2Fdoi.org%5C%2F10.1007%5C%2F978-3-030-27397-2_5%27%3Ehttps%3A%5C%2F%5C%2Fdoi.org%5C%2F10.1007%5C%2F978-3-030-27397-2_5%3C%5C%2Fa%3E%3C%5C%2Fdiv%3E%5Cn%3C%5C%2Fdiv%3E%22%2C%22data%22%3A%7B%22itemType%22%3A%22conferencePaper%22%2C%22title%22%3A%22New%20Wine%20in%20Old%20Bottles%3A%20Chatbots%20in%20Government%22%2C%22creators%22%3A%5B%7B%22creatorType%22%3A%22author%22%2C%22firstName%22%3A%22Colin%22%2C%22lastName%22%3A%22van%20Noordt%22%7D%2C%7B%22creatorType%22%3A%22author%22%2C%22firstName%22%3A%22Gianluca%22%2C%22lastName%22%3A%22Misuraca%22%7D%2C%7B%22creatorType%22%3A%22editor%22%2C%22firstName%22%3A%22Panos%22%2C%22lastName%22%3A%22Panagiotopoulos%22%7D%2C%7B%22creatorType%22%3A%22editor%22%2C%22firstName%22%3A%22Noella%22%2C%22lastName%22%3A%22Edelmann%22%7D%2C%7B%22creatorType%22%3A%22editor%22%2C%22firstName%22%3A%22Olivier%22%2C%22lastName%22%3A%22Glassey%22%7D%2C%7B%22creatorType%22%3A%22editor%22%2C%22firstName%22%3A%22Gianluca%22%2C%22lastName%22%3A%22Misuraca%22%7D%2C%7B%22creatorType%22%3A%22editor%22%2C%22firstName%22%3A%22Peter%22%2C%22lastName%22%3A%22Parycek%22%7D%2C%7B%22creatorType%22%3A%22editor%22%2C%22firstName%22%3A%22Thomas%22%2C%22lastName%22%3A%22Lampoltshammer%22%7D%2C%7B%22creatorType%22%3A%22editor%22%2C%22firstName%22%3A%22Barbara%22%2C%22lastName%22%3A%22Re%22%7D%5D%2C%22abstractNote%22%3A%22Advances%20in%20Artificial%20Intelligence%20technologies%20have%20revived%20the%20interest%20in%20Chatbots%20in%20both%20the%20private%20and%20the%20public%20sector.%20Chatbots%20could%20improve%20public%20service%20delivery%20by%20being%20able%20to%20answer%20frequently%20asked%20questions%20and%20conduct%20transactions%2C%20relieving%20staff%20from%20mundane%20tasks.%20However%2C%20previous%20e-Government%20research%20shows%20that%20the%20adoption%20of%20newer%20technologies%20does%20not%20always%20mean%20public%20services%20get%20improved.%20It%20is%20therefore%20of%20interest%20to%20research%20to%20which%20degree%20newer%2C%20advanced%20technologies%20such%20as%20Chatbots%20are%20able%20to%20improve%2C%20change%20and%20restructure%20public%20service%20delivery.%20This%20paper%20gives%20an%20exploratory%20insight%20using%20desktop%20research%20into%20three%20Chatbots%20currently%20used%20in%20the%20public%20administrations%20of%20Latvia%2C%20Vienna%20and%20Bonn.%20The%20findings%20suggest%20that%20minor%20organisational%20changes%20are%20accompanied%20with%20the%20introduction%20of%20Chatbot-technology%20in%20public%20administrations%2C%20but%20question%20whether%20Chatbots%20are%20able%20to%20transform%20traditional%20services%20to%20digital%2C%20integrated%20public%20service%20transactions.%22%2C%22date%22%3A%222019%22%2C%22proceedingsTitle%22%3A%22Electronic%20Participation%22%2C%22conferenceName%22%3A%22%22%2C%22language%22%3A%22en%22%2C%22DOI%22%3A%2210.1007%5C%2F978-3-030-27397-2_5%22%2C%22ISBN%22%3A%22978-3-030-27397-2%22%2C%22url%22%3A%22%22%2C%22collections%22%3A%5B%22ILXVZG9Z%22%5D%2C%22dateModified%22%3A%222024-03-08T23%3A04%3A06Z%22%7D%7D%2C%7B%22key%22%3A%222DB233F6%22%2C%22library%22%3A%7B%22id%22%3A5575045%7D%2C%22meta%22%3A%7B%22creatorSummary%22%3A%22van%20Noordt%20and%20Misuraca%22%2C%22parsedDate%22%3A%222022-05-14%22%2C%22numChildren%22%3A2%7D%2C%22bib%22%3A%22%3Cdiv%20class%3D%5C%22csl-bib-body%5C%22%20style%3D%5C%22line-height%3A%202%3B%20padding-left%3A%201em%3B%20text-indent%3A-1em%3B%5C%22%3E%5Cn%20%20%3Cdiv%20class%3D%5C%22csl-entry%5C%22%3Evan%20Noordt%2C%20C.%2C%20%26amp%3B%20Misuraca%2C%20G.%20%282022%29.%20Artificial%20intelligence%20for%20the%20public%20sector%3A%20results%20of%20landscaping%20the%20use%20of%20AI%20in%20government%20across%20the%20European%20Union.%20%3Ci%3EGovernment%20Information%20Quarterly%3C%5C%2Fi%3E%2C%20101714.%20%3Ca%20href%3D%27https%3A%5C%2F%5C%2Fdoi.org%5C%2F10.1016%5C%2Fj.giq.2022.101714%27%3Ehttps%3A%5C%2F%5C%2Fdoi.org%5C%2F10.1016%5C%2Fj.giq.2022.101714%3C%5C%2Fa%3E%3C%5C%2Fdiv%3E%5Cn%3C%5C%2Fdiv%3E%22%2C%22data%22%3A%7B%22itemType%22%3A%22journalArticle%22%2C%22title%22%3A%22Artificial%20intelligence%20for%20the%20public%20sector%3A%20results%20of%20landscaping%20the%20use%20of%20AI%20in%20government%20across%20the%20European%20Union%22%2C%22creators%22%3A%5B%7B%22creatorType%22%3A%22author%22%2C%22firstName%22%3A%22Colin%22%2C%22lastName%22%3A%22van%20Noordt%22%7D%2C%7B%22creatorType%22%3A%22author%22%2C%22firstName%22%3A%22Gianluca%22%2C%22lastName%22%3A%22Misuraca%22%7D%5D%2C%22abstractNote%22%3A%22Artificial%20Intelligence%20is%20increasingly%20being%20used%20by%20public%20sector%20organisations.%20Previous%20research%20highlighted%20that%20the%20use%20of%20AI%20technologies%20in%20government%20could%20improve%20policy%20making%20processes%2C%20public%20service%20delivery%20and%20the%20internal%20management%20of%20public%20administrations.%20In%20this%20article%2C%20we%20explore%20to%20which%20extent%20the%20use%20of%20AI%20in%20the%20public%20sector%20impacts%20these%20core%20governance%20functions.%20Findings%20from%20the%20review%20of%20a%20sample%20of%20250%20cases%20across%20the%20European%20Union%2C%20show%20that%20AI%20is%20used%20mainly%20to%20support%20improving%20public%20service%20delivery%2C%20followed%20by%20enhancing%20internal%20management%20and%20only%20in%20a%20limited%20number%20assist%20directly%20or%20indirectly%20policy%20decision-making.%20The%20analysis%20suggests%20that%20different%20types%20of%20AI%20technologies%20and%20applications%20are%20used%20in%20different%20governance%20functions%2C%20highlighting%20the%20need%20to%20further%20in-depth%20investigation%20to%20better%20understand%20the%20role%20and%20impact%20of%20use%20in%20what%20is%20being%20defined%20the%20governance%20%5Cu201cof%2C%20with%20and%20by%20AI%5Cu201d.%22%2C%22date%22%3A%222022-05-14%22%2C%22language%22%3A%22en%22%2C%22DOI%22%3A%2210.1016%5C%2Fj.giq.2022.101714%22%2C%22ISSN%22%3A%220740-624X%22%2C%22url%22%3A%22https%3A%5C%2F%5C%2Fwww.sciencedirect.com%5C%2Fscience%5C%2Farticle%5C%2Fpii%5C%2FS0740624X22000478%22%2C%22collections%22%3A%5B%22ILXVZG9Z%22%5D%2C%22dateModified%22%3A%222024-03-08T23%3A03%3A57Z%22%7D%7D%2C%7B%22key%22%3A%22ZWNE2T2R%22%2C%22library%22%3A%7B%22id%22%3A5575045%7D%2C%22meta%22%3A%7B%22creatorSummary%22%3A%22Androutsopoulou%20et%20al.%22%2C%22parsedDate%22%3A%222019-04-01%22%2C%22numChildren%22%3A1%7D%2C%22bib%22%3A%22%3Cdiv%20class%3D%5C%22csl-bib-body%5C%22%20style%3D%5C%22line-height%3A%202%3B%20padding-left%3A%201em%3B%20text-indent%3A-1em%3B%5C%22%3E%5Cn%20%20%3Cdiv%20class%3D%5C%22csl-entry%5C%22%3EAndroutsopoulou%2C%20A.%2C%20Karacapilidis%2C%20N.%2C%20Loukis%2C%20E.%2C%20%26amp%3B%20Charalabidis%2C%20Y.%20%282019%29.%20Transforming%20the%20communication%20between%20citizens%20and%20government%20through%20AI-guided%20chatbots.%20%3Ci%3EGovernment%20Information%20Quarterly%3C%5C%2Fi%3E%2C%20%3Ci%3E36%3C%5C%2Fi%3E%282%29%2C%20358%26%23×2013%3B367.%20%3Ca%20href%3D%27https%3A%5C%2F%5C%2Fdoi.org%5C%2F10.1016%5C%2Fj.giq.2018.10.001%27%3Ehttps%3A%5C%2F%5C%2Fdoi.org%5C%2F10.1016%5C%2Fj.giq.2018.10.001%3C%5C%2Fa%3E%3C%5C%2Fdiv%3E%5Cn%3C%5C%2Fdiv%3E%22%2C%22data%22%3A%7B%22itemType%22%3A%22journalArticle%22%2C%22title%22%3A%22Transforming%20the%20communication%20between%20citizens%20and%20government%20through%20AI-guided%20chatbots%22%2C%22creators%22%3A%5B%7B%22creatorType%22%3A%22author%22%2C%22firstName%22%3A%22Aggeliki%22%2C%22lastName%22%3A%22Androutsopoulou%22%7D%2C%7B%22creatorType%22%3A%22author%22%2C%22firstName%22%3A%22Nikos%22%2C%22lastName%22%3A%22Karacapilidis%22%7D%2C%7B%22creatorType%22%3A%22author%22%2C%22firstName%22%3A%22Euripidis%22%2C%22lastName%22%3A%22Loukis%22%7D%2C%7B%22creatorType%22%3A%22author%22%2C%22firstName%22%3A%22Yannis%22%2C%22lastName%22%3A%22Charalabidis%22%7D%5D%2C%22abstractNote%22%3A%22Driven%20by%20%5Cu2018success%20stories%5Cu2019%20reported%20by%20private%20sector%20firms%2C%20government%20agencies%20have%20also%20started%20adopting%20various%20Artificial%20Intelligence%20%28AI%29%20technologies%20in%20diverse%20domains%20%28e.g.%20health%2C%20taxation%2C%20and%20education%29%3B%20however%2C%20extensive%20research%20is%20required%20in%20order%20to%20exploit%20the%20full%20potential%20of%20AI%20in%20the%20public%20sector%2C%20and%20leverage%20various%20AI%20technologies%20to%20address%20important%20problems%5C%2Fneeds.%20This%20paper%20makes%20a%20contribution%20in%20this%20direction%3A%20it%20presents%20a%20novel%20approach%2C%20as%20well%20as%20the%20architecture%20of%20an%20ICT%20platform%20supporting%20it%2C%20for%20the%20advanced%20exploitation%20of%20a%20specific%20AI%20technology%2C%20namely%20chatbots%2C%20in%20the%20public%20sector%20in%20order%20to%20address%20a%20crucial%20issue%3A%20the%20improvement%20of%20communication%20between%20government%20and%20citizens%20%28which%20has%20for%20long%20time%20been%20problematic%29.%20The%20proposed%20approach%20builds%20on%20natural%20language%20processing%2C%20machine%20learning%20and%20data%20mining%20technologies%2C%20and%20leverages%20existing%20data%20of%20various%20forms%20%28such%20as%20documents%20containing%20legislation%20and%20directives%2C%20structured%20data%20from%20government%20agencies%27%20operational%20systems%2C%20social%20media%20data%2C%20etc.%29%2C%20in%20order%20to%20develop%20a%20new%20digital%20channel%20of%20communication%20between%20citizens%20and%20government.%20Making%20use%20of%20appropriately%20structured%20and%20semantically%20annotated%20data%2C%20this%20channel%20enables%20%5Cu2018richer%5Cu2019%20and%20more%20expressive%20interaction%20of%20citizens%20with%20government%20in%20everyday%20language%2C%20facilitating%20and%20advancing%20both%20information%20seeking%20and%20conducting%20of%20transactions.%20Compared%20to%20existing%20digital%20channels%2C%20the%20proposed%20approach%20is%20appropriate%20for%20a%20wider%20range%20of%20citizens%27%20interactions%2C%20with%20higher%20levels%20of%20complexity%2C%20ambiguity%20and%20uncertainty.%20In%20close%20co-operation%20with%20three%20Greek%20government%20agencies%20%28the%20Ministry%20of%20Finance%2C%20a%20social%20security%20organization%2C%20and%20a%20big%20local%20government%20organization%29%2C%20this%20approach%20has%20been%20validated%20through%20a%20series%20of%20application%20scenarios.%22%2C%22date%22%3A%222019-04-01%22%2C%22language%22%3A%22%22%2C%22DOI%22%3A%2210.1016%5C%2Fj.giq.2018.10.001%22%2C%22ISSN%22%3A%220740-624X%22%2C%22url%22%3A%22https%3A%5C%2F%5C%2Fwww.sciencedirect.com%5C%2Fscience%5C%2Farticle%5C%2Fpii%5C%2FS0740624X17304008%22%2C%22collections%22%3A%5B%22ILXVZG9Z%22%5D%2C%22dateModified%22%3A%222024-03-08T23%3A03%3A41Z%22%7D%7D%2C%7B%22key%22%3A%22B975SY7C%22%2C%22library%22%3A%7B%22id%22%3A5575045%7D%2C%22meta%22%3A%7B%22creatorSummary%22%3A%22Wang%20et%20al.%22%2C%22parsedDate%22%3A%222022-04-01%22%2C%22numChildren%22%3A1%7D%2C%22bib%22%3A%22%3Cdiv%20class%3D%5C%22csl-bib-body%5C%22%20style%3D%5C%22line-height%3A%202%3B%20padding-left%3A%201em%3B%20text-indent%3A-1em%3B%5C%22%3E%5Cn%20%20%3Cdiv%20class%3D%5C%22csl-entry%5C%22%3EWang%2C%20Y.%2C%20Zhang%2C%20N.%2C%20%26amp%3B%20Zhao%2C%20X.%20%282022%29.%20Understanding%20the%20Determinants%20in%20the%20Different%20Government%20AI%20Adoption%20Stages%3A%20Evidence%20of%20Local%20Government%20Chatbots%20in%20China.%20%3Ci%3ESocial%20Science%20Computer%20Review%3C%5C%2Fi%3E%2C%20%3Ci%3E40%3C%5C%2Fi%3E%282%29%2C%20534%26%23×2013%3B554.%20%3Ca%20href%3D%27https%3A%5C%2F%5C%2Fdoi.org%5C%2F10.1177%5C%2F0894439320980132%27%3Ehttps%3A%5C%2F%5C%2Fdoi.org%5C%2F10.1177%5C%2F0894439320980132%3C%5C%2Fa%3E%3C%5C%2Fdiv%3E%5Cn%3C%5C%2Fdiv%3E%22%2C%22data%22%3A%7B%22itemType%22%3A%22journalArticle%22%2C%22title%22%3A%22Understanding%20the%20Determinants%20in%20the%20Different%20Government%20AI%20Adoption%20Stages%3A%20Evidence%20of%20Local%20Government%20Chatbots%20in%20China%22%2C%22creators%22%3A%5B%7B%22creatorType%22%3A%22author%22%2C%22firstName%22%3A%22Youkui%22%2C%22lastName%22%3A%22Wang%22%7D%2C%7B%22creatorType%22%3A%22author%22%2C%22firstName%22%3A%22Nan%22%2C%22lastName%22%3A%22Zhang%22%7D%2C%7B%22creatorType%22%3A%22author%22%2C%22firstName%22%3A%22Xuejiao%22%2C%22lastName%22%3A%22Zhao%22%7D%5D%2C%22abstractNote%22%3A%22With%20the%20rapid%20development%20of%20artificial%20intelligence%20%28AI%29%2C%20countries%20are%20increasingly%20adopting%20AI-guided%20chatbots%20to%20improve%20service%20on%20government%20portals.%20The%20reduction%20in%20face-to-face%20services%20under%20COVID-19%20pandemic%20will%20further%20accelerate%20this%20trend.%20However%2C%20the%20adoption%20and%20performance%20of%20the%20existing%20chatbots%20differ.%20Based%20on%20the%20literature%20on%20e-government%20adoption%20and%20innovative%20policy%20innovation%20diffusion%2C%20this%20article%20examines%20both%20the%20initial%20and%20postadoption%20stages%20of%20chatbot%20usage%20in%20China%5Cu2019s%20local%20governments.%20While%20the%20first%20phase%20employs%20the%20survival%20model%20of%20event%20history%20analysis%20to%20explore%20the%20factors%20that%20influence%20the%20decision%20to%20adopt%20chatbots%20in%20local%20government%2C%20the%20second%20phase%20analyzes%20the%20determinants%20of%20those%20chatbots%5Cu2019%20performance%20in%20the%20postadoption%20stage.%20We%20find%20that%20pressure%20factors%20and%20readiness%20factors%20play%20different%20roles%20in%20the%20different%20adoption%20stages.%20Although%20pressure%20can%20encourage%20local%20governments%20to%20implement%20chatbots%2C%20these%20governments%5Cu2019%20readiness%20determined%20how%20well%20the%20chatbots%20perform%20after%20their%20initial%20adoption.%20The%20implications%20and%20limitations%20of%20the%20research%20are%20also%20discussed.%22%2C%22date%22%3A%222022-04-01%22%2C%22language%22%3A%22en%22%2C%22DOI%22%3A%2210.1177%5C%2F0894439320980132%22%2C%22ISSN%22%3A%220894-4393%22%2C%22url%22%3A%22https%3A%5C%2F%5C%2Fdoi.org%5C%2F10.1177%5C%2F0894439320980132%22%2C%22collections%22%3A%5B%22ILXVZG9Z%22%5D%2C%22dateModified%22%3A%222024-03-08T23%3A03%3A40Z%22%7D%7D%2C%7B%22key%22%3A%22423AELMK%22%2C%22library%22%3A%7B%22id%22%3A5575045%7D%2C%22meta%22%3A%7B%22creatorSummary%22%3A%22RobotsMali%22%2C%22parsedDate%22%3A%222024%22%2C%22numChildren%22%3A0%7D%2C%22bib%22%3A%22%3Cdiv%20class%3D%5C%22csl-bib-body%5C%22%20style%3D%5C%22line-height%3A%202%3B%20padding-left%3A%201em%3B%20text-indent%3A-1em%3B%5C%22%3E%5Cn%20%20%3Cdiv%20class%3D%5C%22csl-entry%5C%22%3ERobotsMali.%20%282024%29.%20%3Ci%3EAI%20for%20Education%20%26%23×2013%3B%20Centre%20Collaboratif%20pour%20l%26%23×2019%3Beducation%20en%20Robotique%20et%20en%20Intelligence%20Artificielle%3C%5C%2Fi%3E.%20%3Ca%20href%3D%27https%3A%5C%2F%5C%2Frobotsmali.org%5C%2FProjets%5C%2Fai-for-education%5C%2F%27%3Ehttps%3A%5C%2F%5C%2Frobotsmali.org%5C%2FProjets%5C%2Fai-for-education%5C%2F%3C%5C%2Fa%3E%3C%5C%2Fdiv%3E%5Cn%3C%5C%2Fdiv%3E%22%2C%22data%22%3A%7B%22itemType%22%3A%22blogPost%22%2C%22title%22%3A%22AI%20for%20Education%20%5Cu2013%20Centre%20Collaboratif%20pour%20l%27education%20en%20Robotique%20et%20en%20Intelligence%20Artificielle%22%2C%22creators%22%3A%5B%7B%22creatorType%22%3A%22author%22%2C%22firstName%22%3A%22%22%2C%22lastName%22%3A%22RobotsMali%22%7D%5D%2C%22abstractNote%22%3A%22RobotsMali%20est%20le%20Centre%20Collaboratif%20pour%20l%5Cu2019%5Cu00e9ducation%20en%20Robotique%20et%20en%20Intelligence%20Artificielle.%20Sous%20l%5Cu2019%5Cu00e9gide%20du%20Minist%5Cu00e8re%20de%20l%5Cu2019Education%2C%20de%20l%5Cu2019Enseignement%20Sup%5Cu00e9rieure%20et%20de%20la%20Recherche%20Scientifique%2C%20la%20structure%20s%5Cu2019applique%20depuis%20sa%20cr%5Cu00e9ation%20%5Cu00e0%20former%20des%20leaders%20dans%20les%20domaines%20des%20STEM%2C%20de%20la%20robotique%20et%20de%20l%5Cu2019intelligence%20artificielle.%22%2C%22blogTitle%22%3A%22%22%2C%22date%22%3A%222024%22%2C%22url%22%3A%22https%3A%5C%2F%5C%2Frobotsmali.org%5C%2FProjets%5C%2Fai-for-education%5C%2F%22%2C%22language%22%3A%22fr-FR%22%2C%22collections%22%3A%5B%22ILXVZG9Z%22%5D%2C%22dateModified%22%3A%222024-03-08T23%3A03%3A24Z%22%7D%7D%2C%7B%22key%22%3A%22QVY6FGPR%22%2C%22library%22%3A%7B%22id%22%3A5575045%7D%2C%22meta%22%3A%7B%22creatorSummary%22%3A%22Risemberg%20and%20Dosunmu%22%2C%22parsedDate%22%3A%222024-02-28%22%2C%22numChildren%22%3A0%7D%2C%22bib%22%3A%22%3Cdiv%20class%3D%5C%22csl-bib-body%5C%22%20style%3D%5C%22line-height%3A%202%3B%20padding-left%3A%201em%3B%20text-indent%3A-1em%3B%5C%22%3E%5Cn%20%20%3Cdiv%20class%3D%5C%22csl-entry%5C%22%3ERisemberg%2C%20A.%2C%20%26amp%3B%20Dosunmu%2C%20D.%20%282024%2C%20February%2028%29.%20The%20AI%20project%20pushing%20local%20languages%20to%20replace%20French%20in%20Mali%26%23×2019%3Bs%20schools.%20%3Ci%3ERest%20of%20World%3C%5C%2Fi%3E.%20%3Ca%20href%3D%27https%3A%5C%2F%5C%2Frestofworld.org%5C%2F2024%5C%2Fmali-ai-translate-local-language-education%5C%2F%27%3Ehttps%3A%5C%2F%5C%2Frestofworld.org%5C%2F2024%5C%2Fmali-ai-translate-local-language-education%5C%2F%3C%5C%2Fa%3E%3C%5C%2Fdiv%3E%5Cn%3C%5C%2Fdiv%3E%22%2C%22data%22%3A%7B%22itemType%22%3A%22blogPost%22%2C%22title%22%3A%22The%20AI%20project%20pushing%20local%20languages%20to%20replace%20French%20in%20Mali%5Cu2019s%20schools%22%2C%22creators%22%3A%5B%7B%22creatorType%22%3A%22author%22%2C%22firstName%22%3A%22Annie%22%2C%22lastName%22%3A%22Risemberg%22%7D%2C%7B%22creatorType%22%3A%22author%22%2C%22firstName%22%3A%22Damilare%22%2C%22lastName%22%3A%22Dosunmu%22%7D%5D%2C%22abstractNote%22%3A%22RobotsMali%20uses%20ChatGPT%2C%20Google%20Translate%2C%20and%20other%20AI%20tools%20in%20hopes%20of%20helping%20young%20students%20learn%20faster%20and%20stay%20in%20school.%22%2C%22blogTitle%22%3A%22Rest%20of%20World%22%2C%22date%22%3A%222024-02-28T11%3A00%2B00%3A00%22%2C%22url%22%3A%22https%3A%5C%2F%5C%2Frestofworld.org%5C%2F2024%5C%2Fmali-ai-translate-local-language-education%5C%2F%22%2C%22language%22%3A%22en-US%22%2C%22collections%22%3A%5B%22ILXVZG9Z%22%5D%2C%22dateModified%22%3A%222024-03-08T23%3A03%3A24Z%22%7D%7D%2C%7B%22key%22%3A%22TS8JJASQ%22%2C%22library%22%3A%7B%22id%22%3A5575045%7D%2C%22meta%22%3A%7B%22creatorSummary%22%3A%22Choi%20et%20al.%22%2C%22parsedDate%22%3A%222024-02-01%22%2C%22numChildren%22%3A1%7D%2C%22bib%22%3A%22%3Cdiv%20class%3D%5C%22csl-bib-body%5C%22%20style%3D%5C%22line-height%3A%202%3B%20padding-left%3A%201em%3B%20text-indent%3A-1em%3B%5C%22%3E%5Cn%20%20%3Cdiv%20class%3D%5C%22csl-entry%5C%22%3EChoi%2C%20J.%20H.%2C%20Garrod%2C%20O.%2C%20Atherton%2C%20P.%2C%20Joyce-Gibbons%2C%20A.%2C%20Mason-Sesay%2C%20M.%2C%20%26amp%3B%20Bj%26%23xF6%3Brkegren%2C%20D.%20%282024%29.%20%3Ci%3EAre%20LLMs%20Useful%20in%20the%20Poorest%20Schools%3F%20TheTeacher.%20AI%20in%20Sierra%20Leone%3C%5C%2Fi%3E%20%28arXiv%3A2310.02982%29.%20arXiv.%20%3Ca%20href%3D%27https%3A%5C%2F%5C%2Fdoi.org%5C%2F10.48550%5C%2FarXiv.2310.02982%27%3Ehttps%3A%5C%2F%5C%2Fdoi.org%5C%2F10.48550%5C%2FarXiv.2310.02982%3C%5C%2Fa%3E%3C%5C%2Fdiv%3E%5Cn%3C%5C%2Fdiv%3E%22%2C%22data%22%3A%7B%22itemType%22%3A%22preprint%22%2C%22title%22%3A%22Are%20LLMs%20Useful%20in%20the%20Poorest%20Schools%3F%20TheTeacher.%20AI%20in%20Sierra%20Leone%22%2C%22creators%22%3A%5B%7B%22creatorType%22%3A%22author%22%2C%22firstName%22%3A%22Jun%20Ho%22%2C%22lastName%22%3A%22Choi%22%7D%2C%7B%22creatorType%22%3A%22author%22%2C%22firstName%22%3A%22Oliver%22%2C%22lastName%22%3A%22Garrod%22%7D%2C%7B%22creatorType%22%3A%22author%22%2C%22firstName%22%3A%22Paul%22%2C%22lastName%22%3A%22Atherton%22%7D%2C%7B%22creatorType%22%3A%22author%22%2C%22firstName%22%3A%22Andrew%22%2C%22lastName%22%3A%22Joyce-Gibbons%22%7D%2C%7B%22creatorType%22%3A%22author%22%2C%22firstName%22%3A%22Miriam%22%2C%22lastName%22%3A%22Mason-Sesay%22%7D%2C%7B%22creatorType%22%3A%22author%22%2C%22firstName%22%3A%22Daniel%22%2C%22lastName%22%3A%22Bj%5Cu00f6rkegren%22%7D%5D%2C%22abstractNote%22%3A%22Education%20systems%20in%20developing%20countries%20have%20few%20resources%20to%20serve%20large%2C%20poor%20populations.%20How%20might%20generative%20AI%20integrate%20into%20classrooms%3F%20This%20paper%20introduces%20an%20AI%20chatbot%20designed%20to%20assist%20teachers%20in%20Sierra%20Leone%20with%20professional%20development%20to%20improve%20their%20instruction.%20We%20describe%20initial%20findings%20from%20early%20implementation%20across%20122%20schools%20and%20193%20teachers%2C%20and%20analyze%20its%20use%20with%20qualitative%20observations%20and%20by%20analyzing%20queries.%20Teachers%20use%20the%20system%20for%20lesson%20planning%2C%20classroom%20management%2C%20and%20subject%20matter.%20Usage%20is%20sustained%20over%20the%20school%20year%2C%20and%20a%20subset%20of%20teachers%20use%20the%20system%20more%20regularly.%20We%20draw%20conclusions%20from%20these%20findings%20about%20how%20generative%20AI%20systems%20can%20be%20integrated%20into%20school%20systems%20in%20low%20income%20countries.%22%2C%22genre%22%3A%22%22%2C%22repository%22%3A%22arXiv%22%2C%22archiveID%22%3A%22arXiv%3A2310.02982%22%2C%22date%22%3A%222024-02-01%22%2C%22DOI%22%3A%2210.48550%5C%2FarXiv.2310.02982%22%2C%22citationKey%22%3A%22%22%2C%22url%22%3A%22http%3A%5C%2F%5C%2Farxiv.org%5C%2Fabs%5C%2F2310.02982%22%2C%22language%22%3A%22%22%2C%22collections%22%3A%5B%22ILXVZG9Z%22%5D%2C%22dateModified%22%3A%222024-03-08T23%3A03%3A24Z%22%7D%7D%2C%7B%22key%22%3A%22YQ5XQN74%22%2C%22library%22%3A%7B%22id%22%3A5575045%7D%2C%22meta%22%3A%7B%22creatorSummary%22%3A%22Mehrabi%20et%20al.%22%2C%22parsedDate%22%3A%222022-01-25%22%2C%22numChildren%22%3A1%7D%2C%22bib%22%3A%22%3Cdiv%20class%3D%5C%22csl-bib-body%5C%22%20style%3D%5C%22line-height%3A%202%3B%20padding-left%3A%201em%3B%20text-indent%3A-1em%3B%5C%22%3E%5Cn%20%20%3Cdiv%20class%3D%5C%22csl-entry%5C%22%3EMehrabi%2C%20N.%2C%20Morstatter%2C%20F.%2C%20Saxena%2C%20N.%2C%20Lerman%2C%20K.%2C%20%26amp%3B%20Galstyan%2C%20A.%20%282022%29.%20%3Ci%3EA%20Survey%20on%20Bias%20and%20Fairness%20in%20Machine%20Learning%3C%5C%2Fi%3E%20%28arXiv%3A1908.09635%29.%20arXiv.%20%3Ca%20href%3D%27https%3A%5C%2F%5C%2Fdoi.org%5C%2F10.48550%5C%2FarXiv.1908.09635%27%3Ehttps%3A%5C%2F%5C%2Fdoi.org%5C%2F10.48550%5C%2FarXiv.1908.09635%3C%5C%2Fa%3E%3C%5C%2Fdiv%3E%5Cn%3C%5C%2Fdiv%3E%22%2C%22data%22%3A%7B%22itemType%22%3A%22preprint%22%2C%22title%22%3A%22A%20Survey%20on%20Bias%20and%20Fairness%20in%20Machine%20Learning%22%2C%22creators%22%3A%5B%7B%22creatorType%22%3A%22author%22%2C%22firstName%22%3A%22Ninareh%22%2C%22lastName%22%3A%22Mehrabi%22%7D%2C%7B%22creatorType%22%3A%22author%22%2C%22firstName%22%3A%22Fred%22%2C%22lastName%22%3A%22Morstatter%22%7D%2C%7B%22creatorType%22%3A%22author%22%2C%22firstName%22%3A%22Nripsuta%22%2C%22lastName%22%3A%22Saxena%22%7D%2C%7B%22creatorType%22%3A%22author%22%2C%22firstName%22%3A%22Kristina%22%2C%22lastName%22%3A%22Lerman%22%7D%2C%7B%22creatorType%22%3A%22author%22%2C%22firstName%22%3A%22Aram%22%2C%22lastName%22%3A%22Galstyan%22%7D%5D%2C%22abstractNote%22%3A%22With%20the%20widespread%20use%20of%20AI%20systems%20and%20applications%20in%20our%20everyday%20lives%2C%20it%20is%20important%20to%20take%20fairness%20issues%20into%20consideration%20while%20designing%20and%20engineering%20these%20types%20of%20systems.%20Such%20systems%20can%20be%20used%20in%20many%20sensitive%20environments%20to%20make%20important%20and%20life-changing%20decisions%3B%20thus%2C%20it%20is%20crucial%20to%20ensure%20that%20the%20decisions%20do%20not%20reflect%20discriminatory%20behavior%20toward%20certain%20groups%20or%20populations.%20We%20have%20recently%20seen%20work%20in%20machine%20learning%2C%20natural%20language%20processing%2C%20and%20deep%20learning%20that%20addresses%20such%20challenges%20in%20different%20subdomains.%20With%20the%20commercialization%20of%20these%20systems%2C%20researchers%20are%20becoming%20aware%20of%20the%20biases%20that%20these%20applications%20can%20contain%20and%20have%20attempted%20to%20address%20them.%20In%20this%20survey%20we%20investigated%20different%20real-world%20applications%20that%20have%20shown%20biases%20in%20various%20ways%2C%20and%20we%20listed%20different%20sources%20of%20biases%20that%20can%20affect%20AI%20applications.%20We%20then%20created%20a%20taxonomy%20for%20fairness%20definitions%20that%20machine%20learning%20researchers%20have%20defined%20in%20order%20to%20avoid%20the%20existing%20bias%20in%20AI%20systems.%20In%20addition%20to%20that%2C%20we%20examined%20different%20domains%20and%20subdomains%20in%20AI%20showing%20what%20researchers%20have%20observed%20with%20regard%20to%20unfair%20outcomes%20in%20the%20state-of-the-art%20methods%20and%20how%20they%20have%20tried%20to%20address%20them.%20There%20are%20still%20many%20future%20directions%20and%20solutions%20that%20can%20be%20taken%20to%20mitigate%20the%20problem%20of%20bias%20in%20AI%20systems.%20We%20are%20hoping%20that%20this%20survey%20will%20motivate%20researchers%20to%20tackle%20these%20issues%20in%20the%20near%20future%20by%20observing%20existing%20work%20in%20their%20respective%20fields.%22%2C%22genre%22%3A%22%22%2C%22repository%22%3A%22arXiv%22%2C%22archiveID%22%3A%22arXiv%3A1908.09635%22%2C%22date%22%3A%222022-01-25%22%2C%22DOI%22%3A%2210.48550%5C%2FarXiv.1908.09635%22%2C%22citationKey%22%3A%22%22%2C%22url%22%3A%22http%3A%5C%2F%5C%2Farxiv.org%5C%2Fabs%5C%2F1908.09635%22%2C%22language%22%3A%22%22%2C%22collections%22%3A%5B%22ILXVZG9Z%22%5D%2C%22dateModified%22%3A%222024-03-08T23%3A03%3A24Z%22%7D%7D%2C%7B%22key%22%3A%22SA7IH6PZ%22%2C%22library%22%3A%7B%22id%22%3A5575045%7D%2C%22meta%22%3A%7B%22creatorSummary%22%3A%22Castelvecchi%22%2C%22parsedDate%22%3A%222023-06-20%22%2C%22numChildren%22%3A0%7D%2C%22bib%22%3A%22%3Cdiv%20class%3D%5C%22csl-bib-body%5C%22%20style%3D%5C%22line-height%3A%202%3B%20padding-left%3A%201em%3B%20text-indent%3A-1em%3B%5C%22%3E%5Cn%20%20%3Cdiv%20class%3D%5C%22csl-entry%5C%22%3ECastelvecchi%2C%20D.%20%282023%29.%20Open-source%20AI%20chatbots%20are%20booming%20%26%23×2014%3B%20what%20does%20this%20mean%20for%20researchers%3F%20%3Ci%3ENature%3C%5C%2Fi%3E.%20%3Ca%20href%3D%27https%3A%5C%2F%5C%2Fdoi.org%5C%2F10.1038%5C%2Fd41586-023-01970-6%27%3Ehttps%3A%5C%2F%5C%2Fdoi.org%5C%2F10.1038%5C%2Fd41586-023-01970-6%3C%5C%2Fa%3E%3C%5C%2Fdiv%3E%5Cn%3C%5C%2Fdiv%3E%22%2C%22data%22%3A%7B%22itemType%22%3A%22journalArticle%22%2C%22title%22%3A%22Open-source%20AI%20chatbots%20are%20booming%20%5Cu2014%20what%20does%20this%20mean%20for%20researchers%3F%22%2C%22creators%22%3A%5B%7B%22creatorType%22%3A%22author%22%2C%22firstName%22%3A%22Davide%22%2C%22lastName%22%3A%22Castelvecchi%22%7D%5D%2C%22abstractNote%22%3A%22Freely%20accessible%20large%20language%20models%20have%20accelerated%20the%20pace%20of%20innovation%2C%20computer%20scientists%20say.%22%2C%22date%22%3A%222023-06-20%22%2C%22language%22%3A%22en%22%2C%22DOI%22%3A%2210.1038%5C%2Fd41586-023-01970-6%22%2C%22ISSN%22%3A%22%22%2C%22url%22%3A%22https%3A%5C%2F%5C%2Fwww.nature.com%5C%2Farticles%5C%2Fd41586-023-01970-6%22%2C%22collections%22%3A%5B%22CKTAY9YM%22%5D%2C%22dateModified%22%3A%222024-03-08T23%3A01%3A48Z%22%7D%7D%2C%7B%22key%22%3A%22SAAA27EF%22%2C%22library%22%3A%7B%22id%22%3A5575045%7D%2C%22meta%22%3A%7B%22creatorSummary%22%3A%22Ayaz%20et%20al.%22%2C%22parsedDate%22%3A%222023-06-04%22%2C%22numChildren%22%3A1%7D%2C%22bib%22%3A%22%3Cdiv%20class%3D%5C%22csl-bib-body%5C%22%20style%3D%5C%22line-height%3A%202%3B%20padding-left%3A%201em%3B%20text-indent%3A-1em%3B%5C%22%3E%5Cn%20%20%3Cdiv%20class%3D%5C%22csl-entry%5C%22%3EAyaz%2C%20A.%2C%20Nawalgaria%2C%20A.%2C%20%26amp%3B%20Yin%2C%20R.%20%282023%29.%20%3Ci%3ETaught%20by%20the%20Internet%2C%20Exploring%20Bias%20in%20OpenAIs%20GPT3%3C%5C%2Fi%3E%20%28arXiv%3A2306.02428%3B%20Version%201%29.%20arXiv.%20%3Ca%20href%3D%27https%3A%5C%2F%5C%2Fdoi.org%5C%2F10.48550%5C%2FarXiv.2306.02428%27%3Ehttps%3A%5C%2F%5C%2Fdoi.org%5C%2F10.48550%5C%2FarXiv.2306.02428%3C%5C%2Fa%3E%3C%5C%2Fdiv%3E%5Cn%3C%5C%2Fdiv%3E%22%2C%22data%22%3A%7B%22itemType%22%3A%22preprint%22%2C%22title%22%3A%22Taught%20by%20the%20Internet%2C%20Exploring%20Bias%20in%20OpenAIs%20GPT3%22%2C%22creators%22%3A%5B%7B%22creatorType%22%3A%22author%22%2C%22firstName%22%3A%22Ali%22%2C%22lastName%22%3A%22Ayaz%22%7D%2C%7B%22creatorType%22%3A%22author%22%2C%22firstName%22%3A%22Aditya%22%2C%22lastName%22%3A%22Nawalgaria%22%7D%2C%7B%22creatorType%22%3A%22author%22%2C%22firstName%22%3A%22Ruilian%22%2C%22lastName%22%3A%22Yin%22%7D%5D%2C%22abstractNote%22%3A%22This%20research%20delves%20into%20the%20current%20literature%20on%20bias%20in%20Natural%20Language%20Processing%20Models%20and%20the%20techniques%20proposed%20to%20mitigate%20the%20problem%20of%20bias%2C%20including%20why%20it%20is%20important%20to%20tackle%20bias%20in%20the%20first%20place.%20Additionally%2C%20these%20techniques%20are%20further%20analysed%20in%20the%20light%20of%20newly%20developed%20models%20that%20tower%20in%20size%20over%20past%20editions.%20To%20achieve%20those%20aims%2C%20the%20authors%20of%20this%20paper%20conducted%20their%20research%20on%20GPT3%20by%20OpenAI%2C%20the%20largest%20NLP%20model%20available%20to%20consumers%20today.%20With%20175%20billion%20parameters%20in%20contrast%20to%20BERTs%20340%20million%2C%20GPT3%20is%20the%20perfect%20model%20to%20test%20the%20common%20pitfalls%20of%20NLP%20models.%20Tests%20were%20conducted%20through%20the%20development%20of%20an%20Applicant%20Tracking%20System%20using%20GPT3.%20For%20the%20sake%20of%20feasibility%20and%20time%20constraints%2C%20the%20tests%20primarily%20focused%20on%20gender%20bias%2C%20rather%20than%20all%20or%20multiple%20types%20of%20bias.%20Finally%2C%20current%20mitigation%20techniques%20are%20considered%20and%20tested%20to%20measure%20their%20degree%20of%20functionality.%22%2C%22genre%22%3A%22%22%2C%22repository%22%3A%22arXiv%22%2C%22archiveID%22%3A%22arXiv%3A2306.02428%22%2C%22date%22%3A%222023-06-04%22%2C%22DOI%22%3A%2210.48550%5C%2FarXiv.2306.02428%22%2C%22citationKey%22%3A%22%22%2C%22url%22%3A%22http%3A%5C%2F%5C%2Farxiv.org%5C%2Fabs%5C%2F2306.02428%22%2C%22language%22%3A%22%22%2C%22collections%22%3A%5B%22CP3VFVLB%22%5D%2C%22dateModified%22%3A%222024-02-02T16%3A04%3A51Z%22%7D%7D%2C%7B%22key%22%3A%22TEI9JP8K%22%2C%22library%22%3A%7B%22id%22%3A5575045%7D%2C%22meta%22%3A%7B%22creatorSummary%22%3A%22Muldoon%20and%20Wu%22%2C%22parsedDate%22%3A%222023-12-15%22%2C%22numChildren%22%3A1%7D%2C%22bib%22%3A%22%3Cdiv%20class%3D%5C%22csl-bib-body%5C%22%20style%3D%5C%22line-height%3A%202%3B%20padding-left%3A%201em%3B%20text-indent%3A-1em%3B%5C%22%3E%5Cn%20%20%3Cdiv%20class%3D%5C%22csl-entry%5C%22%3EMuldoon%2C%20J.%2C%20%26amp%3B%20Wu%2C%20B.%20A.%20%282023%29.%20Artificial%20Intelligence%20in%20the%20Colonial%20Matrix%20of%20Power.%20%3Ci%3EPhilosophy%20%26amp%3B%20Technology%3C%5C%2Fi%3E%2C%20%3Ci%3E36%3C%5C%2Fi%3E%284%29%2C%2080.%20%3Ca%20href%3D%27https%3A%5C%2F%5C%2Fdoi.org%5C%2F10.1007%5C%2Fs13347-023-00687-8%27%3Ehttps%3A%5C%2F%5C%2Fdoi.org%5C%2F10.1007%5C%2Fs13347-023-00687-8%3C%5C%2Fa%3E%3C%5C%2Fdiv%3E%5Cn%3C%5C%2Fdiv%3E%22%2C%22data%22%3A%7B%22itemType%22%3A%22journalArticle%22%2C%22title%22%3A%22Artificial%20Intelligence%20in%20the%20Colonial%20Matrix%20of%20Power%22%2C%22creators%22%3A%5B%7B%22creatorType%22%3A%22author%22%2C%22firstName%22%3A%22James%22%2C%22lastName%22%3A%22Muldoon%22%7D%2C%7B%22creatorType%22%3A%22author%22%2C%22firstName%22%3A%22Boxi%20A.%22%2C%22lastName%22%3A%22Wu%22%7D%5D%2C%22abstractNote%22%3A%22Drawing%20on%20the%20analytic%20of%20the%20%5Cu201ccolonial%20matrix%20of%20power%5Cu201d%20developed%20by%20An%5Cu00edbal%20Quijano%20within%20the%20Latin%20American%20modernity%5C%2Fcoloniality%20research%20program%2C%20this%20article%20theorises%20how%20a%20system%20of%5Cu00a0coloniality%5Cu00a0underpins%20the%20structuring%20logic%20of%20artificial%20intelligence%20%28AI%29%20systems.%20We%20develop%20a%20framework%20for%20critiquing%20the%20regimes%20of%20global%20labour%20exploitation%20and%20knowledge%20extraction%20that%20are%20rendered%20invisible%20through%20discourses%20of%20the%20purported%20universality%20and%20objectivity%20of%20AI.%20%5Cu200b%5Cu200bThrough%20bringing%20the%20political%20economy%20literature%20on%20AI%20production%20into%20conversation%20with%20scholarly%20work%20on%20decolonial%20AI%20and%20the%20modernity%5C%2Fcoloniality%20research%20program%2C%20we%20advance%20three%20main%20arguments.%20First%2C%20the%20global%20economic%20and%20political%20power%20imbalances%20in%20AI%20production%20are%20inextricably%20linked%20to%20the%20continuities%20of%20historical%20colonialism%2C%20constituting%20the%20colonial%20supply%20chain%20of%20AI.%20Second%2C%20this%20is%20produced%20through%20an%20international%20division%20of%20digital%20labour%20that%20extracts%20value%20from%20majority%20world%20labour%20for%20the%20benefit%20of%20Western%20technology%20companies.%20Third%2C%20this%20perpetuates%20hegemonic%20knowledge%20production%20through%20Western%20values%20and%20knowledge%20that%20marginalises%20non-Western%20alternatives%20within%20AI%5Cu2019s%20production%20and%20limits%20the%20possibilities%20for%20decolonising%20AI.%20By%20locating%20the%20production%20of%20AI%20systems%20within%20the%20colonial%20matrix%20of%20power%2C%20we%20contribute%20to%20critical%20and%20decolonial%20literature%20on%20the%20legacies%20of%20colonialism%20in%20AI%20and%20the%20hierarchies%20of%20power%20and%20extraction%20that%20shape%20the%20development%20of%20AI%20today.%22%2C%22date%22%3A%222023-12-15%22%2C%22language%22%3A%22en%22%2C%22DOI%22%3A%2210.1007%5C%2Fs13347-023-00687-8%22%2C%22ISSN%22%3A%222210-5441%22%2C%22url%22%3A%22https%3A%5C%2F%5C%2Fdoi.org%5C%2F10.1007%5C%2Fs13347-023-00687-8%22%2C%22collections%22%3A%5B%22B6PTFKKB%22%2C%22LK5NK5YI%22%5D%2C%22dateModified%22%3A%222024-01-26T15%3A33%3A37Z%22%7D%7D%2C%7B%22key%22%3A%22ASHBUC8U%22%2C%22library%22%3A%7B%22id%22%3A5575045%7D%2C%22meta%22%3A%7B%22creatorSummary%22%3A%22UNDESA%22%2C%22parsedDate%22%3A%222023%22%2C%22numChildren%22%3A0%7D%2C%22bib%22%3A%22%3Cdiv%20class%3D%5C%22csl-bib-body%5C%22%20style%3D%5C%22line-height%3A%202%3B%20padding-left%3A%201em%3B%20text-indent%3A-1em%3B%5C%22%3E%5Cn%20%20%3Cdiv%20class%3D%5C%22csl-entry%5C%22%3EUNDESA.%20%282023%29.%20%3Ci%3EE-Government%20Development%20Index%3C%5C%2Fi%3E.%20%3Ca%20href%3D%27https%3A%5C%2F%5C%2Fpublicadministration.un.org%5C%2Fegovkb%5C%2Fen-us%5C%2FAbout%5C%2FOverview%5C%2F-E-Government-Development-Index%27%3Ehttps%3A%5C%2F%5C%2Fpublicadministration.un.org%5C%2Fegovkb%5C%2Fen-us%5C%2FAbout%5C%2FOverview%5C%2F-E-Government-Development-Index%3C%5C%2Fa%3E%3C%5C%2Fdiv%3E%5Cn%3C%5C%2Fdiv%3E%22%2C%22data%22%3A%7B%22itemType%22%3A%22webpage%22%2C%22title%22%3A%22E-Government%20Development%20Index%22%2C%22creators%22%3A%5B%7B%22creatorType%22%3A%22author%22%2C%22firstName%22%3A%22%22%2C%22lastName%22%3A%22UNDESA%22%7D%5D%2C%22abstractNote%22%3A%22%22%2C%22date%22%3A%222023%22%2C%22url%22%3A%22https%3A%5C%2F%5C%2Fpublicadministration.un.org%5C%2Fegovkb%5C%2Fen-us%5C%2FAbout%5C%2FOverview%5C%2F-E-Government-Development-Index%22%2C%22language%22%3A%22%22%2C%22collections%22%3A%5B%22ZKFMLJUC%22%5D%2C%22dateModified%22%3A%222023-12-15T15%3A06%3A09Z%22%7D%7D%2C%7B%22key%22%3A%22GYEC9525%22%2C%22library%22%3A%7B%22id%22%3A5575045%7D%2C%22meta%22%3A%7B%22creatorSummary%22%3A%22Nemani%20et%20al.%22%2C%22parsedDate%22%3A%222023-06%22%2C%22numChildren%22%3A1%7D%2C%22bib%22%3A%22%3Cdiv%20class%3D%5C%22csl-bib-body%5C%22%20style%3D%5C%22line-height%3A%202%3B%20padding-left%3A%201em%3B%20text-indent%3A-1em%3B%5C%22%3E%5Cn%20%20%3Cdiv%20class%3D%5C%22csl-entry%5C%22%3ENemani%2C%20P.%2C%20Joel%2C%20Y.%20D.%2C%20Vijay%2C%20P.%2C%20%26amp%3B%20Liza%2C%20F.%20F.%20%282023%29.%20%3Ci%3EGender%20Bias%20in%20Transformer%20Models%3A%20A%20comprehensive%20survey%3C%5C%2Fi%3E.%20arXiv.%3C%5C%2Fdiv%3E%5Cn%3C%5C%2Fdiv%3E%22%2C%22data%22%3A%7B%22itemType%22%3A%22document%22%2C%22title%22%3A%22Gender%20Bias%20in%20Transformer%20Models%3A%20A%20comprehensive%20survey%22%2C%22creators%22%3A%5B%7B%22creatorType%22%3A%22author%22%2C%22firstName%22%3A%22Praneeth%22%2C%22lastName%22%3A%22Nemani%22%7D%2C%7B%22creatorType%22%3A%22author%22%2C%22firstName%22%3A%22Yericherla%20Deepak%22%2C%22lastName%22%3A%22Joel%22%7D%2C%7B%22creatorType%22%3A%22author%22%2C%22firstName%22%3A%22Palla%22%2C%22lastName%22%3A%22Vijay%22%7D%2C%7B%22creatorType%22%3A%22author%22%2C%22firstName%22%3A%22Farhana%20Ferdousi%22%2C%22lastName%22%3A%22Liza%22%7D%5D%2C%22abstractNote%22%3A%22Gender%20bias%20in%20artificial%20intelligence%20%28AI%29%20has%20emerged%20as%20a%20pressing%20concern%20with%20profound%20implications%20for%20individuals%27%20lives.%20This%20paper%20presents%20a%20comprehensive%20survey%20that%20explores%20gender%20bias%20in%20Transformer%20models%20from%20a%20linguistic%20perspective.%20While%20the%20existence%20of%20gender%20bias%20in%20language%20models%20has%20been%20acknowledged%20in%20previous%20studies%2C%20there%20remains%20a%20lack%20of%20consensus%20on%20how%20to%20effectively%20measure%20and%20evaluate%20this%20bias.%20Our%20survey%20critically%20examines%20the%20existing%20literature%20on%20gender%20bias%20in%20Transformers%2C%20shedding%20light%20on%20the%20diverse%20methodologies%20and%20metrics%20employed%20to%20assess%20bias.%20Several%20limitations%20in%20current%20approaches%20to%20measuring%20gender%20bias%20in%20Transformers%20are%20identified%2C%20encompassing%20the%20utilization%20of%20incomplete%20or%20flawed%20metrics%2C%20inadequate%20dataset%20sizes%2C%20and%20a%20dearth%20of%20standardization%20in%20evaluation%20methods.%20Furthermore%2C%20our%20survey%20delves%20into%20the%20potential%20ramifications%20of%20gender%20bias%20in%20Transformers%20for%20downstream%20applications%2C%20including%20dialogue%20systems%20and%20machine%20translation.%20We%20underscore%20the%20importance%20of%20fostering%20equity%20and%20fairness%20in%20these%20systems%20by%20emphasizing%20the%20need%20for%20heightened%20awareness%20and%20accountability%20in%20developing%20and%20deploying%20language%20technologies.%20This%20paper%20serves%20as%20a%20comprehensive%20overview%20of%20gender%20bias%20in%20Transformer%20models%2C%20providing%20novel%20insights%20and%20offering%20valuable%20directions%20for%20future%20research%20in%20this%20critical%20domain.%22%2C%22date%22%3A%222023-06%22%2C%22language%22%3A%22%22%2C%22url%22%3A%22%22%2C%22collections%22%3A%5B%2268SB3MLX%22%2C%22JDIALZLQ%22%5D%2C%22dateModified%22%3A%222023-09-12T10%3A55%3A50Z%22%7D%7D%2C%7B%22key%22%3A%22RX76KXJA%22%2C%22library%22%3A%7B%22id%22%3A5575045%7D%2C%22meta%22%3A%7B%22creatorSummary%22%3A%22Saldanha%20et%20al.%22%2C%22parsedDate%22%3A%222022-02-12%22%2C%22numChildren%22%3A0%7D%2C%22bib%22%3A%22%3Cdiv%20class%3D%5C%22csl-bib-body%5C%22%20style%3D%5C%22line-height%3A%202%3B%20padding-left%3A%201em%3B%20text-indent%3A-1em%3B%5C%22%3E%5Cn%20%20%3Cdiv%20class%3D%5C%22csl-entry%5C%22%3ESaldanha%2C%20D.%20M.%20F.%2C%20Dias%2C%20C.%20N.%2C%20%26amp%3B%20Guillaumon%2C%20S.%20%282022%29.%20Transparency%20and%20accountability%20in%20digital%20public%20services%3A%20Learning%20from%20the%20Brazilian%20cases.%20%3Ci%3EGovernment%20Information%20Quarterly%3C%5C%2Fi%3E%2C%20101680.%20%3Ca%20href%3D%27https%3A%5C%2F%5C%2Fdoi.org%5C%2F10.1016%5C%2Fj.giq.2022.101680%27%3Ehttps%3A%5C%2F%5C%2Fdoi.org%5C%2F10.1016%5C%2Fj.giq.2022.101680%3C%5C%2Fa%3E%3C%5C%2Fdiv%3E%5Cn%3C%5C%2Fdiv%3E%22%2C%22data%22%3A%7B%22itemType%22%3A%22journalArticle%22%2C%22title%22%3A%22Transparency%20and%20accountability%20in%20digital%20public%20services%3A%20Learning%20from%20the%20Brazilian%20cases%22%2C%22creators%22%3A%5B%7B%22creatorType%22%3A%22author%22%2C%22firstName%22%3A%22Douglas%20Morgan%20Fullin%22%2C%22lastName%22%3A%22Saldanha%22%7D%2C%7B%22creatorType%22%3A%22author%22%2C%22firstName%22%3A%22Cleidson%20Nogueira%22%2C%22lastName%22%3A%22Dias%22%7D%2C%7B%22creatorType%22%3A%22author%22%2C%22firstName%22%3A%22Siegrid%22%2C%22lastName%22%3A%22Guillaumon%22%7D%5D%2C%22abstractNote%22%3A%22The%20transparency%20and%20accountability%20of%20systems%20and%20algorithms%20aims%20to%20protect%20the%20user%20against%20undesirable%20or%20harmful%20results%20and%20to%20ensure%20the%20application%20of%20laws%20appropriate%20to%20digital%20environments.%20Thus%2C%20the%20objective%20of%20this%20study%20is%20to%20evaluate%20the%20transparency%20and%20accountability%20provided%20to%20citizens%20in%20three%20of%20the%20main%20digital%20public%20services%20%28e-services%29%20offered%20by%20the%20federal%20administration%20of%20Brazil%20%28ComprasNet%2C%20Sisu%20and%20Naturalizar-se%29%20locally%20recognized%20for%20their%20significant%20relevance%20and%20stage%20of%20development%20and%20use.%20Services%20were%20evaluated%20from%20eight%20perspectives%3A%20accessibility%3B%20awareness%3B%20access%20and%20repair%3B%20accountability%3B%20explanation%3B%20origin%20of%20data%2C%20privacy%20and%20fairness%3B%20auditing%3B%20validation%2C%20accuracy%20and%20testing.%20Adopting%20a%20qualitative%20approach%20through%20comparative%20case%20studies%2C%20this%20research%20contributes%20to%20information%20practices%20theory%20%28construction%20of%20a%20model%20for%20assessing%20transparency%20and%20accountability%20in%20digital%20public%20services%29.%20The%20results%20obtained%20show%20the%20need%20to%20inform%20the%20user%20of%20possible%20bias%20and%20damage%20arising%20from%20these%20systems%2C%20which%20are%20not%20readily%20perceived%3B%20just%20as%20the%20need%20to%20clarify%20the%20benefits%20that%20arise%20from%20the%20collection%20of%20private%20data%20are%20not.%20This%20shows%20that%20computational%20models%20can%20be%20distorted%20as%20a%20result%20of%20biases%20contained%20in%20their%20input%20data%2C%20or%20algorithms.%20This%20paper%20contributes%20through%20an%20innovative%20combination%20of%20dimensions%2C%20as%20a%20tool%20to%20evaluate%20transparency%20and%20accountability%20of%20government%20services.%22%2C%22date%22%3A%22February%2012%2C%202022%22%2C%22language%22%3A%22en%22%2C%22DOI%22%3A%2210.1016%5C%2Fj.giq.2022.101680%22%2C%22ISSN%22%3A%220740-624X%22%2C%22url%22%3A%22https%3A%5C%2F%5C%2Fwww.sciencedirect.com%5C%2Fscience%5C%2Farticle%5C%2Fpii%5C%2FS0740624X22000132%22%2C%22collections%22%3A%5B%22ZKFMLJUC%22%5D%2C%22dateModified%22%3A%222022-02-13T20%3A03%3A57Z%22%7D%7D%2C%7B%22key%22%3A%22F94GQ7ZK%22%2C%22library%22%3A%7B%22id%22%3A5575045%7D%2C%22meta%22%3A%7B%22creatorSummary%22%3A%22Matheus%20et%20al.%22%2C%22parsedDate%22%3A%222021-01-01%22%2C%22numChildren%22%3A1%7D%2C%22bib%22%3A%22%3Cdiv%20class%3D%5C%22csl-bib-body%5C%22%20style%3D%5C%22line-height%3A%202%3B%20padding-left%3A%201em%3B%20text-indent%3A-1em%3B%5C%22%3E%5Cn%20%20%3Cdiv%20class%3D%5C%22csl-entry%5C%22%3EMatheus%2C%20R.%2C%20Janssen%2C%20M.%2C%20%26amp%3B%20Janowski%2C%20T.%20%282021%29.%20Design%20principles%20for%20creating%20digital%20transparency%20in%20government.%20%3Ci%3EGovernment%20Information%20Quarterly%3C%5C%2Fi%3E%2C%20%3Ci%3E38%3C%5C%2Fi%3E%281%29%2C%20101550.%20%3Ca%20href%3D%27https%3A%5C%2F%5C%2Fdoi.org%5C%2F10%5C%2Fgjmz77%27%3Ehttps%3A%5C%2F%5C%2Fdoi.org%5C%2F10%5C%2Fgjmz77%3C%5C%2Fa%3E%3C%5C%2Fdiv%3E%5Cn%3C%5C%2Fdiv%3E%22%2C%22data%22%3A%7B%22itemType%22%3A%22journalArticle%22%2C%22title%22%3A%22Design%20principles%20for%20creating%20digital%20transparency%20in%20government%22%2C%22creators%22%3A%5B%7B%22creatorType%22%3A%22author%22%2C%22firstName%22%3A%22Ricardo%22%2C%22lastName%22%3A%22Matheus%22%7D%2C%7B%22creatorType%22%3A%22author%22%2C%22firstName%22%3A%22Marijn%22%2C%22lastName%22%3A%22Janssen%22%7D%2C%7B%22creatorType%22%3A%22author%22%2C%22firstName%22%3A%22Tomasz%22%2C%22lastName%22%3A%22Janowski%22%7D%5D%2C%22abstractNote%22%3A%22Under%20pressure%20to%20fight%20corruption%2C%20hold%20public%20officials%20accountable%2C%20and%20build%20trust%20with%20citizens%2C%20many%20governments%20pursue%20the%20quest%20for%20greater%20transparency.%20They%20publish%20data%20about%20their%20internal%20operations%2C%20externalize%20decision-making%20processes%2C%20establish%20digital%20inquiry%20lines%20to%20public%20officials%2C%20and%20employ%20other%20forms%20of%20transparency%20using%20digital%20means.%20Despite%20the%20presence%20of%20many%20transparency-enhancing%20digital%20tools%2C%20putting%20such%20tools%20together%20to%20achieve%20the%20desired%20level%20of%20digital%20transparency%2C%20to%20design%20entire%20government%20systems%20for%20digital%20transparency%2C%20remains%20challenging.%20Design%20principles%20and%20other%20design%20guides%20are%20lacking%20in%20this%20area.%20This%20article%20aims%20to%20fill%20this%20gap.%20We%20identify%20a%20set%20of%20barriers%20to%20digital%20transparency%20in%20government%2C%20define%2016%20design%20principles%20to%20overcome%20such%20barriers%2C%20and%20evaluate%20these%20principles%20using%20three%20case%20studies%20from%20different%20countries.%20Some%20principles%20apply%20to%20projects%2C%20others%20to%20systems%2C%20yet%20others%20to%20entire%20organizations.%20To%20achieve%20digital%20transparency%2C%20before%20building%20and%20deploying%20digital%20solutions%2C%20government%20organizations%20should%20build%20technological%20and%20institutional%20foundations%20and%20use%20such%20foundations%20to%20organize%20themselves%20for%20transparency.%20The%20proposed%20design%20principles%20can%20help%20develop%20and%20apply%20such%20foundations.%22%2C%22date%22%3A%22January%201%2C%202021%22%2C%22language%22%3A%22en%22%2C%22DOI%22%3A%2210%5C%2Fgjmz77%22%2C%22ISSN%22%3A%220740-624X%22%2C%22url%22%3A%22https%3A%5C%2F%5C%2Fwww.sciencedirect.com%5C%2Fscience%5C%2Farticle%5C%2Fpii%5C%2FS0740624X20303294%22%2C%22collections%22%3A%5B%22ZKFMLJUC%22%5D%2C%22dateModified%22%3A%222021-05-27T21%3A38%3A22Z%22%7D%7D%2C%7B%22key%22%3A%22TFWCQ8PK%22%2C%22library%22%3A%7B%22id%22%3A5575045%7D%2C%22meta%22%3A%7B%22creatorSummary%22%3A%22Zambrano%22%2C%22parsedDate%22%3A%222017-12%22%2C%22numChildren%22%3A1%7D%2C%22bib%22%3A%22%3Cdiv%20class%3D%5C%22csl-bib-body%5C%22%20style%3D%5C%22line-height%3A%202%3B%20padding-left%3A%201em%3B%20text-indent%3A-1em%3B%5C%22%3E%5Cn%20%20%3Cdiv%20class%3D%5C%22csl-entry%5C%22%3EZambrano%2C%20R.%20%282017%29.%20%3Ci%3EUnpacking%20the%20disruptive%20potential%20of%20blockchain%20technology%20for%20human%20development.%3C%5C%2Fi%3E%20IDRC.%20%3Ca%20href%3D%27https%3A%5C%2F%5C%2Fwww.idrc.ca%5C%2Fen%5C%2Fstories%5C%2Fblockchain%27%3Ehttps%3A%5C%2F%5C%2Fwww.idrc.ca%5C%2Fen%5C%2Fstories%5C%2Fblockchain%3C%5C%2Fa%3E%3C%5C%2Fdiv%3E%5Cn%3C%5C%2Fdiv%3E%22%2C%22data%22%3A%7B%22itemType%22%3A%22report%22%2C%22title%22%3A%22Unpacking%20the%20disruptive%20potential%20of%20blockchain%20technology%20for%20human%20development.%22%2C%22creators%22%3A%5B%7B%22creatorType%22%3A%22author%22%2C%22firstName%22%3A%22Raul%22%2C%22lastName%22%3A%22Zambrano%22%7D%5D%2C%22abstractNote%22%3A%22Unpacking%20the%20disruptive%20potential%20of%20blockchain%20technology%20for%20human%20development.%22%2C%22reportNumber%22%3A%22%22%2C%22reportType%22%3A%22%22%2C%22institution%22%3A%22IDRC%22%2C%22date%22%3A%22Dec%202017%22%2C%22language%22%3A%22en%22%2C%22url%22%3A%22https%3A%5C%2F%5C%2Fwww.idrc.ca%5C%2Fen%5C%2Fstories%5C%2Fblockchain%22%2C%22collections%22%3A%5B%22MM7XK3ZH%22%5D%2C%22dateModified%22%3A%222020-08-26T13%3A47%3A28Z%22%7D%7D%2C%7B%22key%22%3A%222Z46M5YY%22%2C%22library%22%3A%7B%22id%22%3A5575045%7D%2C%22meta%22%3A%7B%22creatorSummary%22%3A%22Janowski%22%2C%22parsedDate%22%3A%222015-07-01%22%2C%22numChildren%22%3A1%7D%2C%22bib%22%3A%22%3Cdiv%20class%3D%5C%22csl-bib-body%5C%22%20style%3D%5C%22line-height%3A%202%3B%20padding-left%3A%201em%3B%20text-indent%3A-1em%3B%5C%22%3E%5Cn%20%20%3Cdiv%20class%3D%5C%22csl-entry%5C%22%3EJanowski%2C%20T.%20%282015%29.%20Digital%20government%20evolution%3A%20From%20transformation%20to%20contextualization.%20%3Ci%3EGovernment%20Information%20Quarterly%3C%5C%2Fi%3E%2C%20%3Ci%3E32%3C%5C%2Fi%3E%283%29%2C%20221%26%23×2013%3B236.%20%3Ca%20href%3D%27https%3A%5C%2F%5C%2Fdoi.org%5C%2F10.1016%5C%2Fj.giq.2015.07.001%27%3Ehttps%3A%5C%2F%5C%2Fdoi.org%5C%2F10.1016%5C%2Fj.giq.2015.07.001%3C%5C%2Fa%3E%3C%5C%2Fdiv%3E%5Cn%3C%5C%2Fdiv%3E%22%2C%22data%22%3A%7B%22itemType%22%3A%22journalArticle%22%2C%22title%22%3A%22Digital%20government%20evolution%3A%20From%20transformation%20to%20contextualization%22%2C%22creators%22%3A%5B%7B%22creatorType%22%3A%22author%22%2C%22firstName%22%3A%22Tomasz%22%2C%22lastName%22%3A%22Janowski%22%7D%5D%2C%22abstractNote%22%3A%22The%20Digital%20Government%20landscape%20is%20continuously%20changing%20to%20reflect%20how%20governments%20are%20trying%20to%20find%20innovative%20digital%20solutions%20to%20social%2C%20economic%2C%20political%20and%20other%20pressures%2C%20and%20how%20they%20transform%20themselves%20in%20the%20process.%20Understanding%20and%20predicting%20such%20changes%20is%20important%20for%20policymakers%2C%20government%20executives%2C%20researchers%20and%20all%20those%20who%20prepare%2C%20make%2C%20implement%20or%20evaluate%20Digital%20Government%20decisions.%20This%20article%20argues%20that%20the%20concept%20of%20Digital%20Government%20evolves%20toward%20more%20complexity%20and%20greater%20contextualization%20and%20specialization%2C%20similar%20to%20evolution-like%20processes%20that%20lead%20to%20changes%20in%20cultures%20and%20societies.%20To%20this%20end%2C%20the%20article%20presents%20a%20four-stage%20Digital%20Government%20Evolution%20Model%20comprising%20Digitization%20%28Technology%20in%20Government%29%2C%20Transformation%20%28Electronic%20Government%29%2C%20Engagement%20%28Electronic%20Governance%29%20and%20Contextualization%20%28Policy-Driven%20Electronic%20Governance%29%20stages%3B%20provides%20some%20evidence%20in%20support%20of%20this%20model%20drawing%20upon%20the%20study%20of%20the%20Digital%20Government%20literature%20published%20in%20Government%20Information%20Quarterly%20between%201992%20and%202014%3B%20and%20presents%20a%20Digital%20Government%20Stage%20Analysis%20Framework%20to%20explain%20the%20evolution.%20As%20the%20article%20consolidates%20a%20representative%20body%20of%20the%20Digital%20Government%20literature%2C%20it%20could%20be%20also%20used%20for%20defining%20and%20integrating%20future%20research%20in%20the%20area.%22%2C%22date%22%3A%22July%201%2C%202015%22%2C%22language%22%3A%22%22%2C%22DOI%22%3A%2210.1016%5C%2Fj.giq.2015.07.001%22%2C%22ISSN%22%3A%220740-624X%22%2C%22url%22%3A%22http%3A%5C%2F%5C%2Fwww.sciencedirect.com%5C%2Fscience%5C%2Farticle%5C%2Fpii%5C%2FS0740624X15000775%22%2C%22collections%22%3A%5B%22ZKFMLJUC%22%5D%2C%22dateModified%22%3A%222019-03-31T12%3A34%3A55Z%22%7D%7D%5D%7D
Cortés-Cediel, M. E., Segura-Tinoco, A., Cantador, I., & Rodríguez Bolívar, M. P. (2023). Trends and challenges of e-government chatbots: Advances in exploring open government data and citizen participation content.
Government Information Quarterly,
40(4), 101877.
https://doi.org/10.1016/j.giq.2023.101877
Alon-Barkat, S., & Busuioc, M. (2023). Human–AI Interactions in Public Sector Decision Making: “Automation Bias” and “Selective Adherence” to Algorithmic Advice.
Journal of Public Administration Research and Theory,
33(1), 153–169.
https://doi.org/10.1093/jopart/muac007
Maragno, G., Tangi, L., Gastaldi, L., & Benedetti, M. (2023). AI as an organizational agent to nurture: effectively introducing chatbots in public entities.
Public Management Review,
25(11), 2135–2165.
https://doi.org/10.1080/14719037.2022.2063935
Medaglia, R., & Tangi, L. (2022). The adoption of Artificial Intelligence in the public sector in Europe: drivers, features, and impacts.
Proceedings of the 15th International Conference on Theory and Practice of Electronic Governance, 10–18.
https://doi.org/10.1145/3560107.3560110
Valle-Cruz, D., García-Contreras, R., & Gil-Garcia, J. R. (2023). Exploring the negative impacts of artificial intelligence in government: the dark side of intelligent algorithms and cognitive machines.
International Review of Administrative Sciences, 00208523231187051.
https://doi.org/10.1177/00208523231187051
Medaglia, R., Gil-Garcia, J. R., & Pardo, T. A. (2023). Artificial Intelligence in Government: Taking Stock and Moving Forward.
Social Science Computer Review,
41(1), 123–140.
https://doi.org/10.1177/08944393211034087
van Noordt, C., & Misuraca, G. (2019). New Wine in Old Bottles: Chatbots in Government. In P. Panagiotopoulos, N. Edelmann, O. Glassey, G. Misuraca, P. Parycek, T. Lampoltshammer, & B. Re (Eds.),
Electronic Participation (pp. 49–59). Springer International Publishing.
https://doi.org/10.1007/978-3-030-27397-2_5
van Noordt, C., & Misuraca, G. (2022). Artificial intelligence for the public sector: results of landscaping the use of AI in government across the European Union.
Government Information Quarterly, 101714.
https://doi.org/10.1016/j.giq.2022.101714
Androutsopoulou, A., Karacapilidis, N., Loukis, E., & Charalabidis, Y. (2019). Transforming the communication between citizens and government through AI-guided chatbots.
Government Information Quarterly,
36(2), 358–367.
https://doi.org/10.1016/j.giq.2018.10.001
Wang, Y., Zhang, N., & Zhao, X. (2022). Understanding the Determinants in the Different Government AI Adoption Stages: Evidence of Local Government Chatbots in China.
Social Science Computer Review,
40(2), 534–554.
https://doi.org/10.1177/0894439320980132
RobotsMali. (2024).
AI for Education – Centre Collaboratif pour l’education en Robotique et en Intelligence Artificielle.
https://robotsmali.org/Projets/ai-for-education/
Risemberg, A., & Dosunmu, D. (2024, February 28). The AI project pushing local languages to replace French in Mali’s schools.
Rest of World.
https://restofworld.org/2024/mali-ai-translate-local-language-education/
Choi, J. H., Garrod, O., Atherton, P., Joyce-Gibbons, A., Mason-Sesay, M., & Björkegren, D. (2024).
Are LLMs Useful in the Poorest Schools? TheTeacher. AI in Sierra Leone (arXiv:2310.02982). arXiv.
https://doi.org/10.48550/arXiv.2310.02982
Mehrabi, N., Morstatter, F., Saxena, N., Lerman, K., & Galstyan, A. (2022).
A Survey on Bias and Fairness in Machine Learning (arXiv:1908.09635). arXiv.
https://doi.org/10.48550/arXiv.1908.09635
Castelvecchi, D. (2023). Open-source AI chatbots are booming — what does this mean for researchers?
Nature.
https://doi.org/10.1038/d41586-023-01970-6
Ayaz, A., Nawalgaria, A., & Yin, R. (2023).
Taught by the Internet, Exploring Bias in OpenAIs GPT3 (arXiv:2306.02428; Version 1). arXiv.
https://doi.org/10.48550/arXiv.2306.02428
Muldoon, J., & Wu, B. A. (2023). Artificial Intelligence in the Colonial Matrix of Power.
Philosophy & Technology,
36(4), 80.
https://doi.org/10.1007/s13347-023-00687-8
Nemani, P., Joel, Y. D., Vijay, P., & Liza, F. F. (2023). Gender Bias in Transformer Models: A comprehensive survey. arXiv.
Saldanha, D. M. F., Dias, C. N., & Guillaumon, S. (2022). Transparency and accountability in digital public services: Learning from the Brazilian cases.
Government Information Quarterly, 101680.
https://doi.org/10.1016/j.giq.2022.101680
Matheus, R., Janssen, M., & Janowski, T. (2021). Design principles for creating digital transparency in government.
Government Information Quarterly,
38(1), 101550.
https://doi.org/10/gjmz77
Zambrano, R. (2017).
Unpacking the disruptive potential of blockchain technology for human development. IDRC.
https://www.idrc.ca/en/stories/blockchain
Janowski, T. (2015). Digital government evolution: From transformation to contextualization.
Government Information Quarterly,
32(3), 221–236.
https://doi.org/10.1016/j.giq.2015.07.001
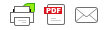