AI’s astounding evolution in the last decade has been nothing less than spectacular, pace doomers. It has undoubtedly exceeded most expectations, bringing numerous benefits and generating new challenges and risks. The latter is crucial to understand as AI has a bi-polar personality. It is indeed friend and foe. It all depends on how humans (ab)use it. However, in the current global governance environment and structures, only a few seem to have a say in how that is done. The rest of us are sitting on the fence at best. That said, there is no reason why AI should have a different role when invited to join the global disinformation ball. Deploying the three analytical categories mentioned in the first part of this post (data, output, scope), we can identify three AI phases associated with disinformation production and dissemination.
The first phase saw AI play as a Sweeper. Supported by the refinement of Neural Networks (NNs) algorithms and the lauded success of Deep Learning (DL), Machine Learning’s victory lap was cheered universally. Supervised and unsupervised learning models were the most common, with the first reporting the most expansive success. AI could successfully process images and identify objects and subjects with unheard accuracy for the first time in history. The same was the case for voice and other recording types. Indeed, AI could now see us and even recognize our faces, thus raising further privacy challenges. Big data played a critical role in this development. Outputs, however, were limited to prediction, trend analysis, classification, and pattern identification. And while the new algorithms were powerful, their scope was limited to specific tasks or sectors. In this phase, AI was deployed as a helpful tool to detect disinformation, thus playing the role of a staunch defender while being attacked from all flanks. Historically, this phase started in 2012.
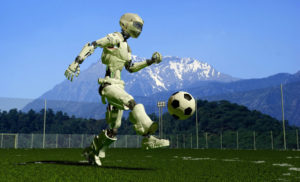
In the second phase, which started in late 2016, AI did a 180 and became an incisive Striker. The advance of Reinforcement Learning (RL) and the emergence of new algorithms such as GANs (Generative Adversarial Networks) and early Transformer algorithms showed that the new AI flavors could create data independently using incentive and feedback mechanisms built into the algorithms to optimize outputs. AlphaGo and AlphaZero are successful examples here. On the other hand, GANs and similar algorithms gave birth to deepfakes. In that light, AI could now be deployed to manipulate data and generate new information and images with fantastic accuracy, following the principle that new outputs should have the same statistical properties as the data used to train them. However, doing away with such guidance did not break the algorithms. In any case, AI became part of the attacking disinformation team, thereby in direct competition with AI Sweepers, who now had yet another striker to deal with – and one with the sharpest accuracy. In this phase, big data was not the only player in town, as the new AI could create it. Moreover, AI could generate new digital products, challenging to differentiate from those based on reality – even by AI. However, AI’s scope remained limited to specific domains or sectors.
The launching of ChatGPT 11 months ago ushered in a new phase where AI now assumed the role of a Coach, capable of managing sweepers and strikers. The maturation of Transformer algorithms led to the emergence of GPTs (Generative Pre-trained Transformers) shaped as LLMs (Large language models), of which ChatGPT is still the best example. Such models use Supervised, Semi-supervised, and Unsupervised learning and RL algorithms with a human touch (labeled as RL with Human Feedback, RLHF) as part of their training process. In this phase, Big data regained most of the ground lost in the second phase. Nevertheless, the critical change can be captured under the output and scope headings. Unlike its recent ancestors, LLMs can create a vast array of new outputs while their scope is now cross-cutting, thus including multiple domains and sectors. Not surprisingly, this set of models is seen as AGI (Artificial General Intelligence) in its early infancy. In any event, their impact on disinformation is less clear than previous and less powerful AI models. Recent research suggests their adverse effects have been overblown as the disinformation realm is already quite complex and sophisticated. It could go either way or work simultaneously, but more research is needed to make a final call. The Coach needs a Team Manager, that is for sure.
Note that such research could benefit substantially by using LLMs and other AI. For example, AI could be instrumental in expediting the elaboration of literature reviews and bibliometric analysis. Similarly, LLMs could be used to translate into English research and papers published in other languages while providing succinct summaries of each. That would also facilitate a more contextual approach to disinformation production and distribution. By the same token, Open Source LLMs could be fine-tuned and aligned to relevant domain-specific areas of knowledge, such as disinformation and AI. Researchers in these areas do not need to ask the model to summarize the Zero Law of Thermodynamics. Finally, AI could also be deployed to handle a gamut of administrative tasks that usually consume precious time of researchers in the Global South, where academic environments are still evolving.
In sum, AI has multiple roles to play regarding disinformation. But whatever that is, it will not solve the issues once and for all. After all, disinformation is a governance disease that digital technologies can ameliorate or aggravate but are not the antidote to its poisonous venom.
Raúl